2024 North American Summer Meeting: June, 2024
Information Aggregation in Directed Networks
Christopher Brown and Svetlana Pevnitskaya
Individuals often can obtain more information by observing previous decisions of others. We present a framework of individual choice under uncertainty for agents connected via a directed network which allows for observational learning. The decision is made once, thus learning from repetition is not possible. We outline properties of networks that affect accuracy of individual choice and information aggregation. Performance is evaluated using two criteria: individual (final agent) and social (group) choice accuracy, with the result that network properties that enhance performance under one
criterion reduce performance under the other. We test theory by designing an experiment with two treatment variables: (1) network structure and (2) method of assigning subjects to positions within a network. In all treatments, there is efficiency loss compared to a benchmark with all Bayesian agents. On average individuals understand the value of observational learning, however there is heterogeneity in the willingness to pay for later positions. In networks with endogenous assignment, the advantage of later positions is eroded as a result of self-selection of more rational subjects to those positions, and greater noise in choices of subjects in earlier positions. Persons exhibit a propensity to overweight information inferred from observed actions of others as opposed to own private signal, which is increasing in the number of observed actions.
Preview
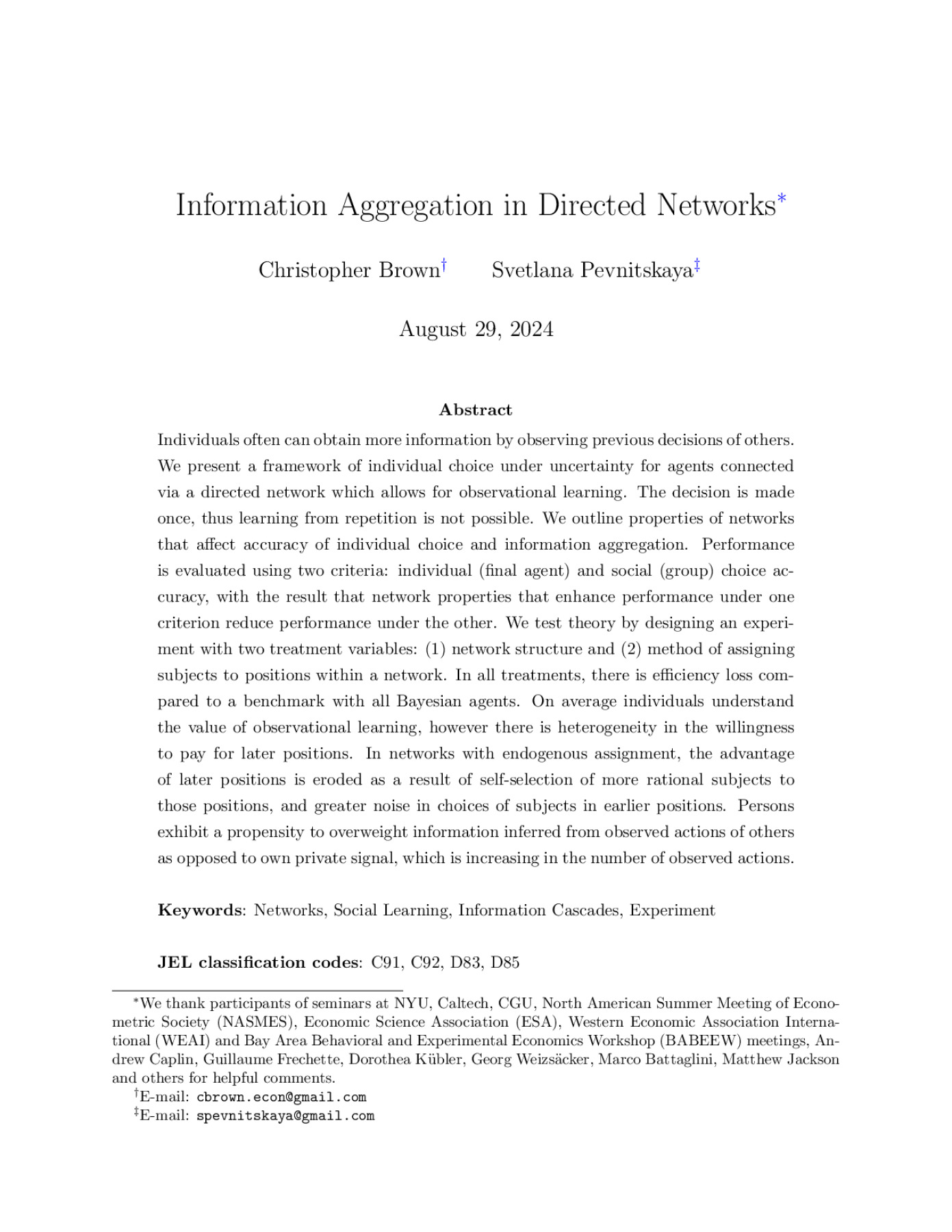
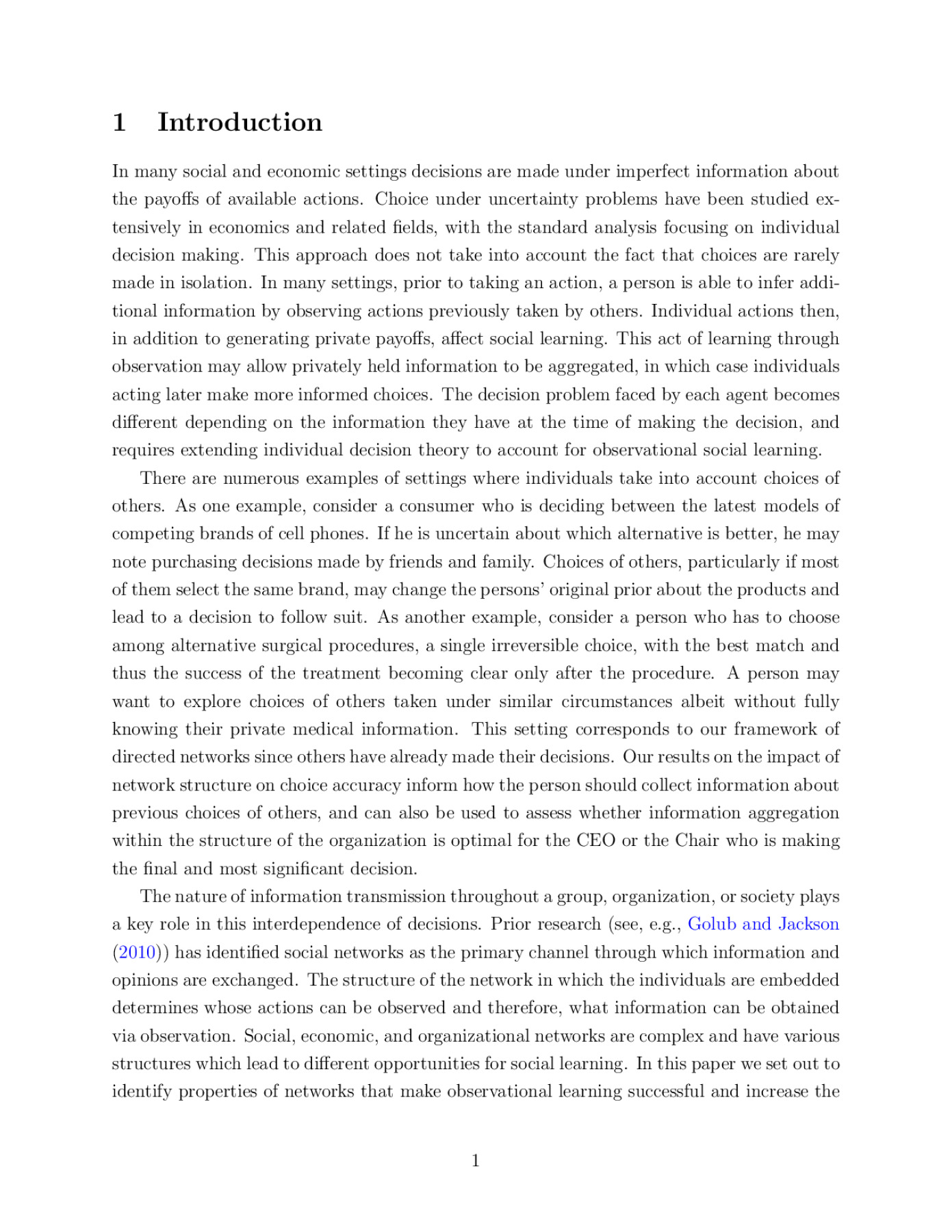
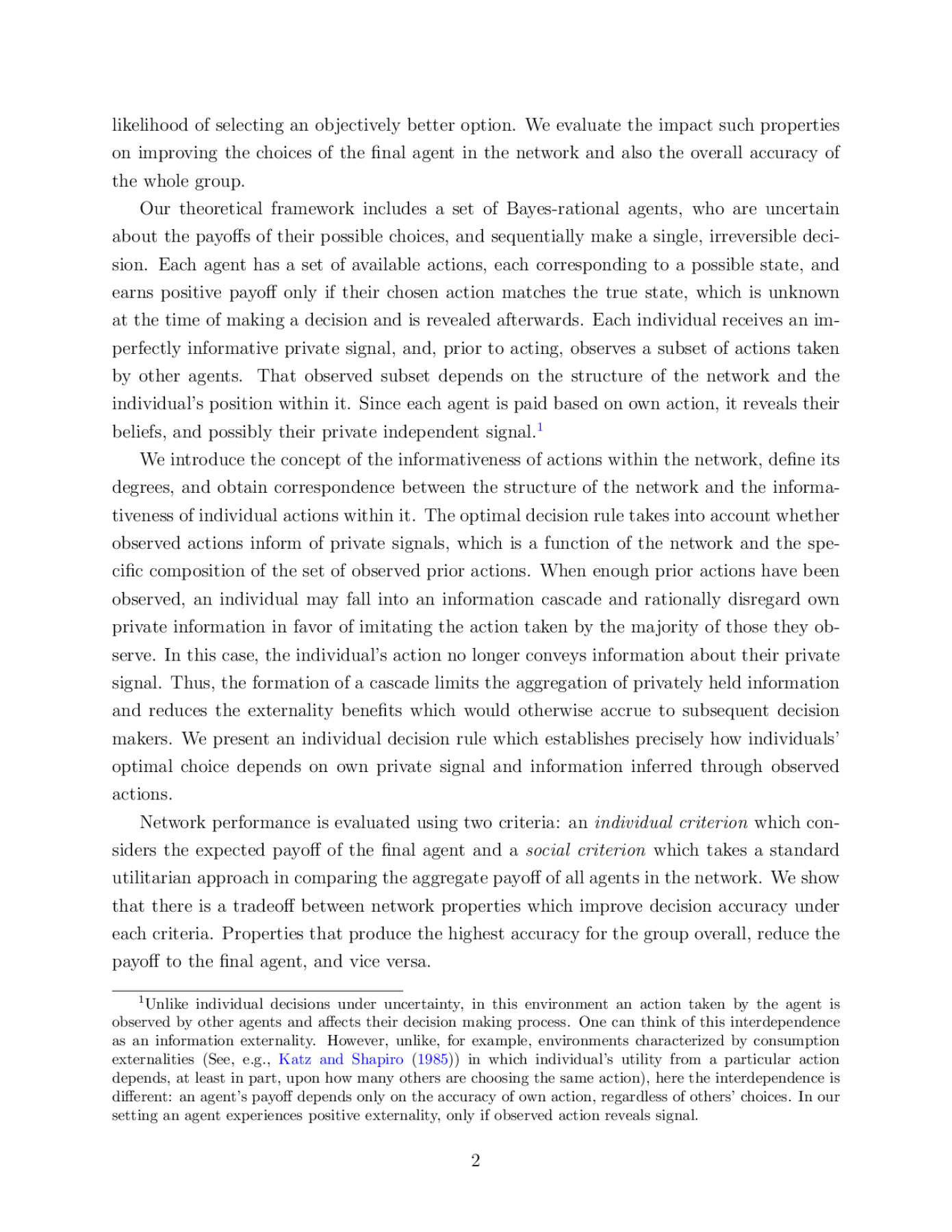
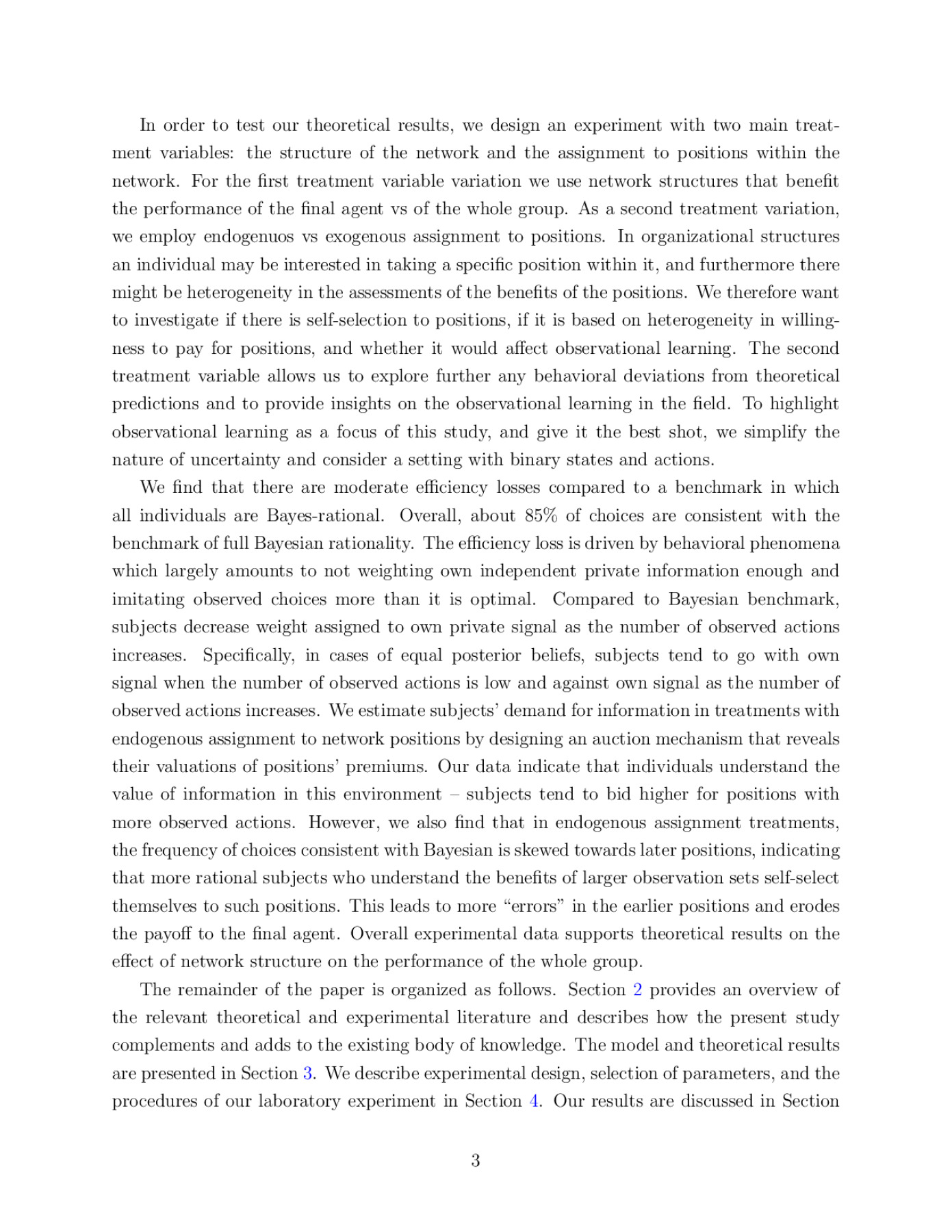
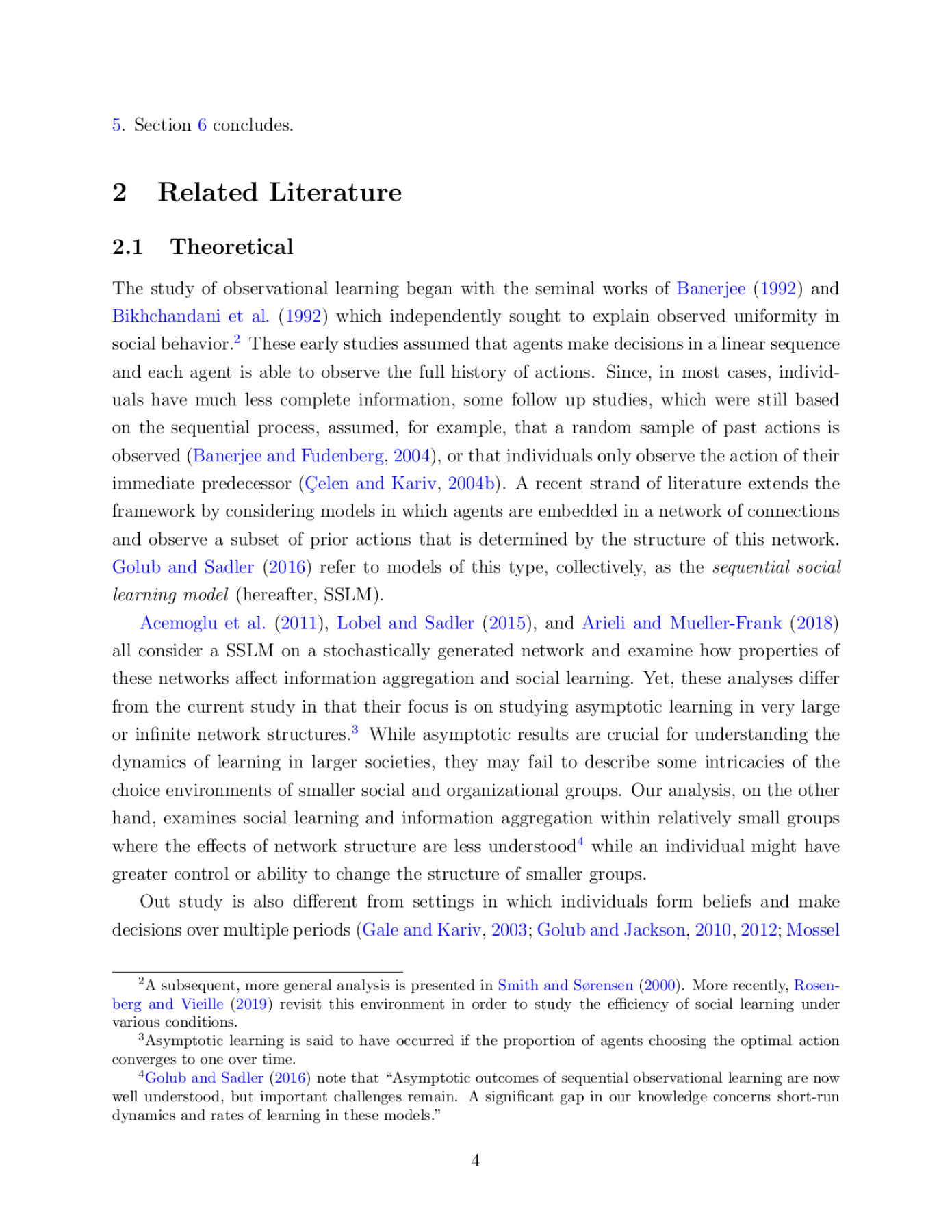
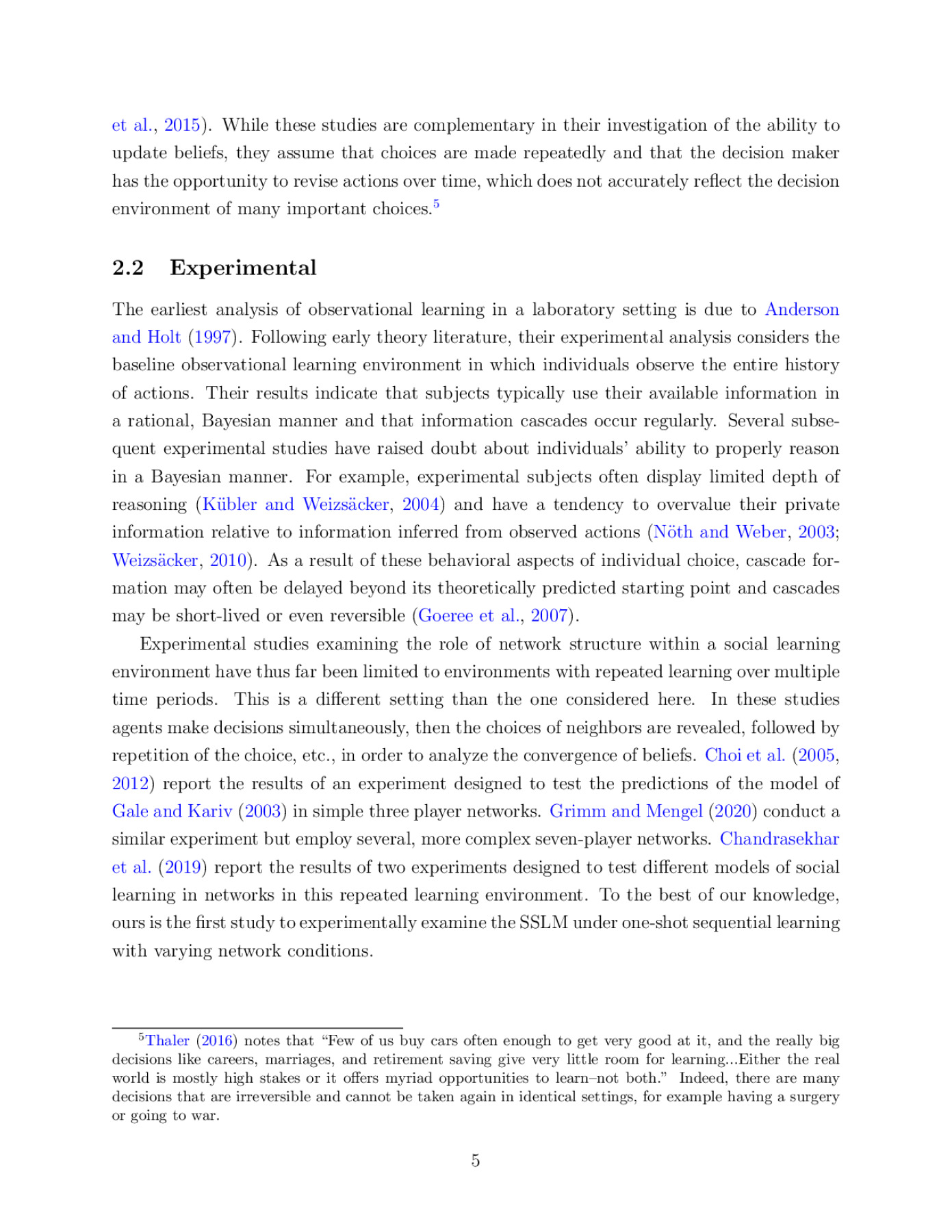
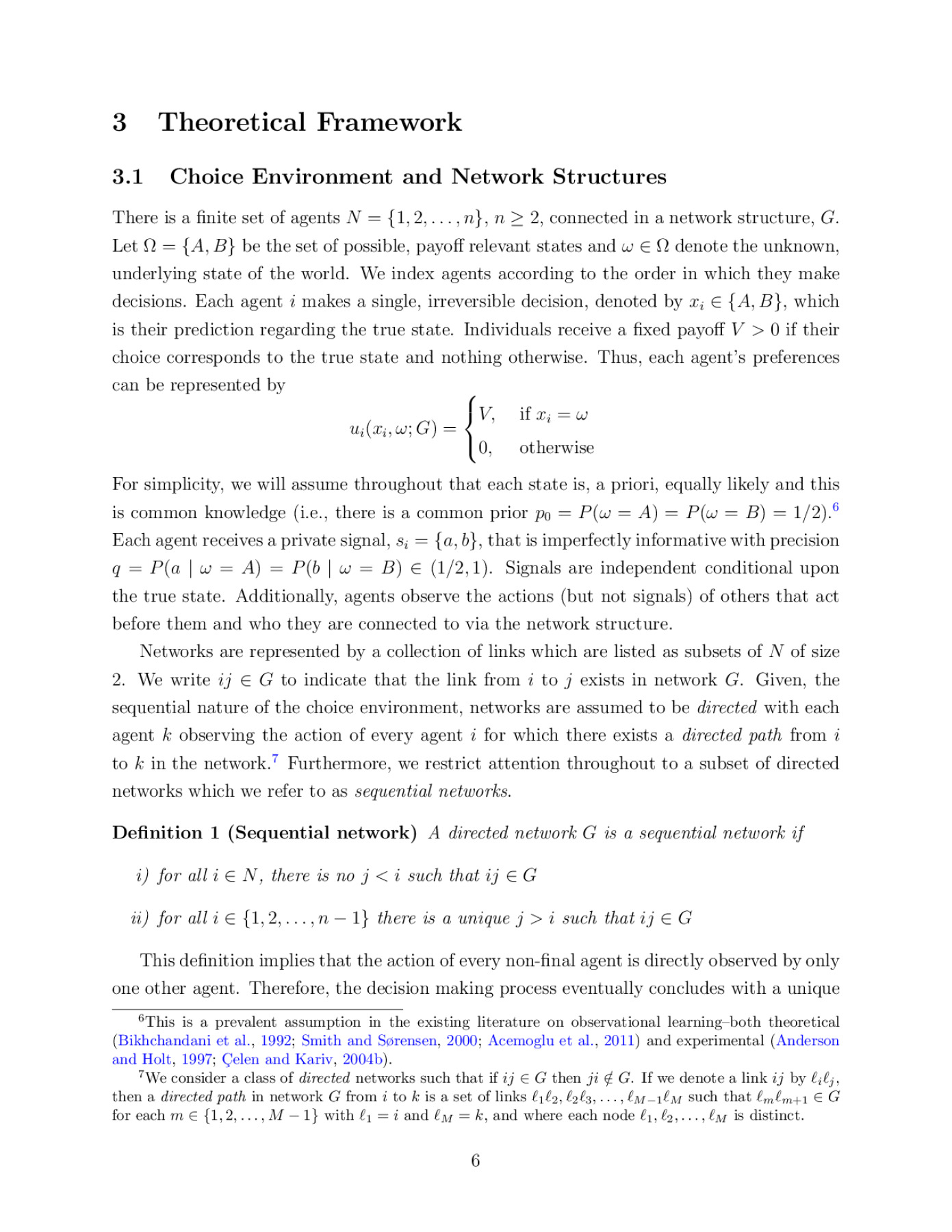
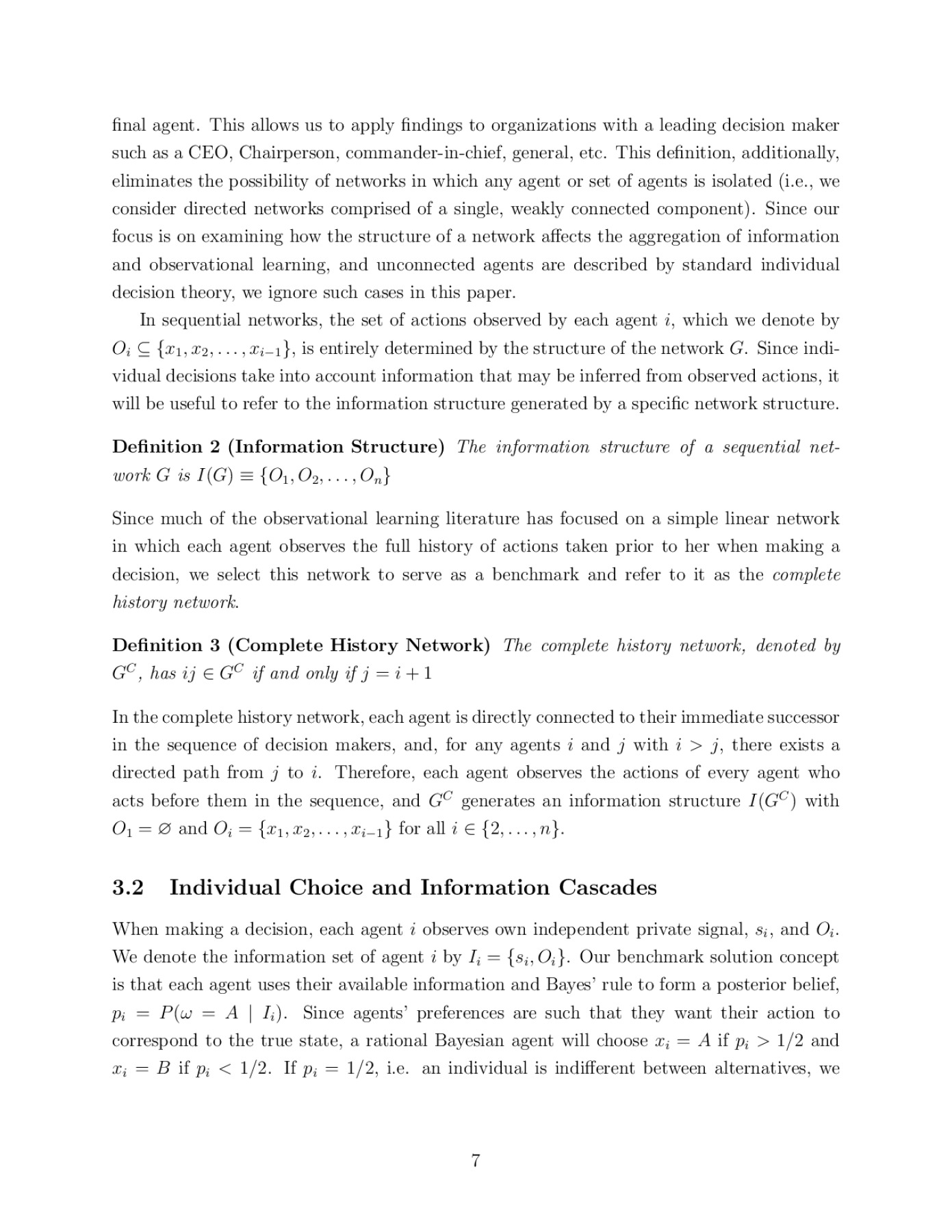
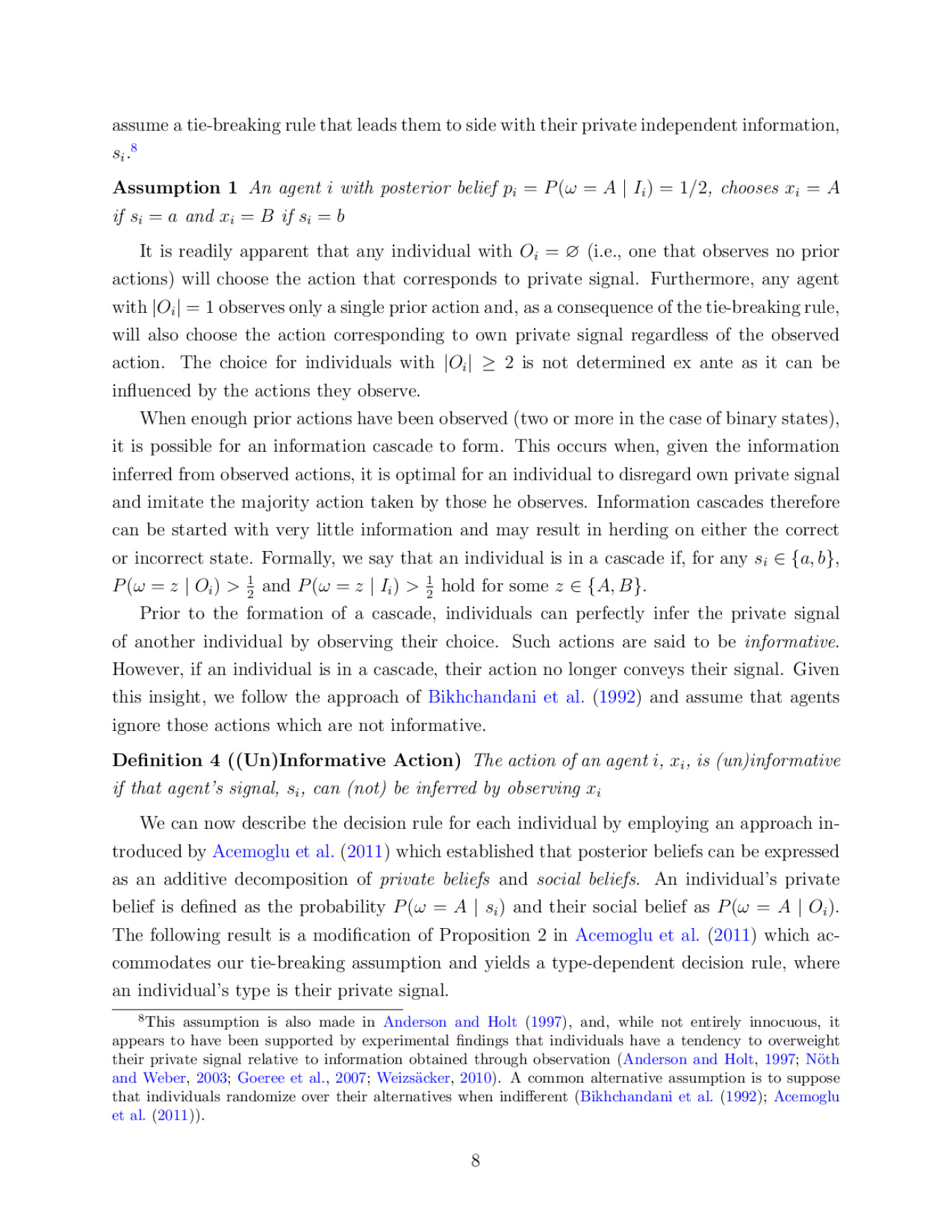
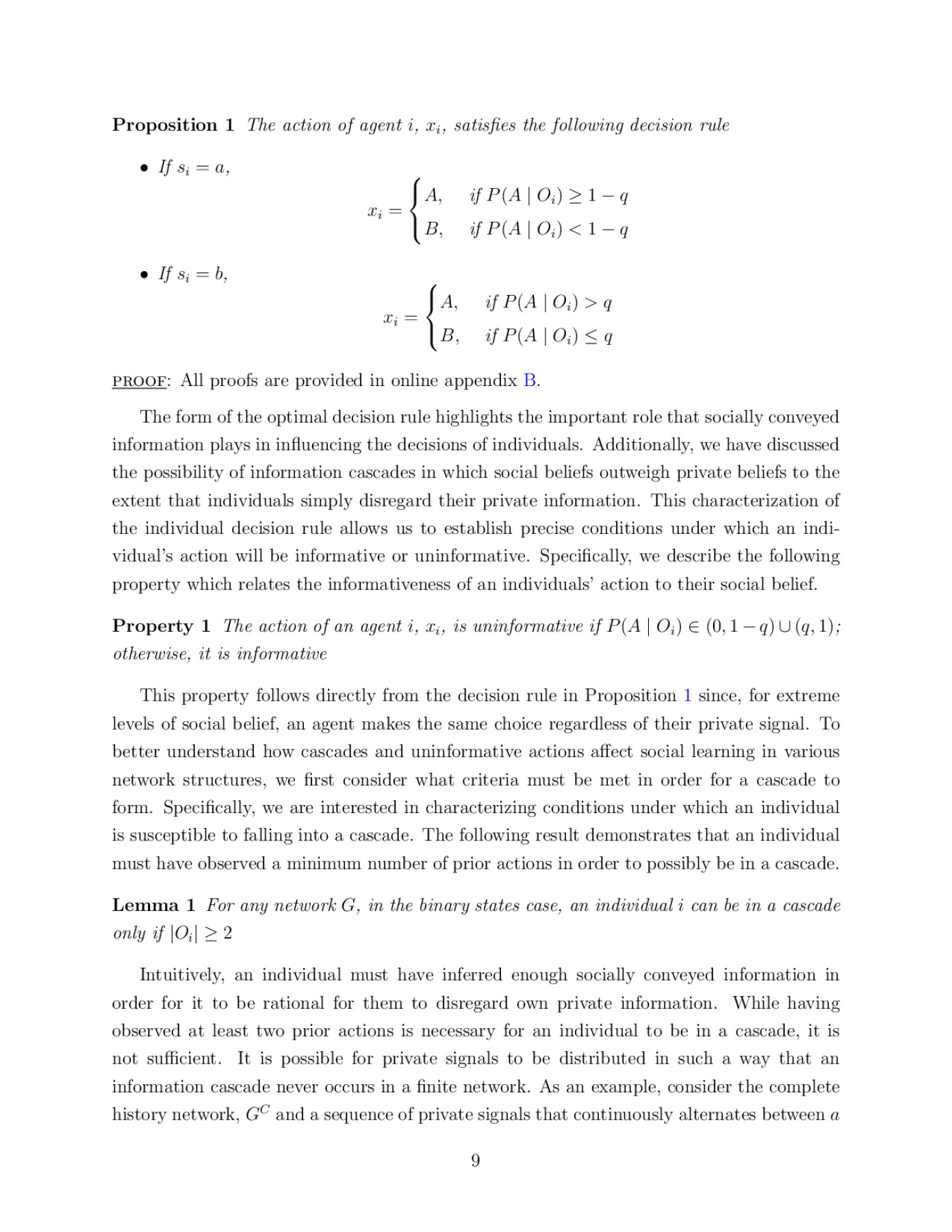

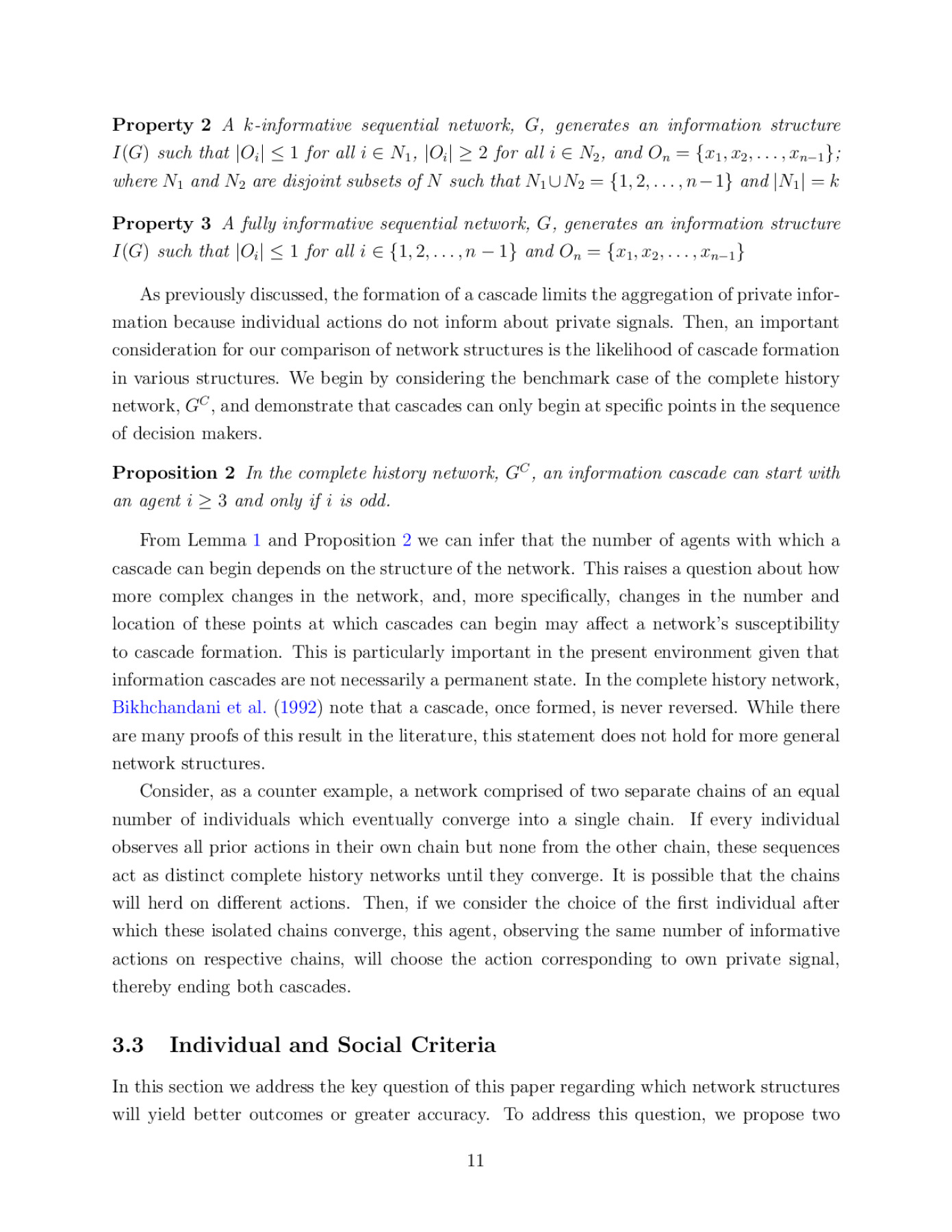
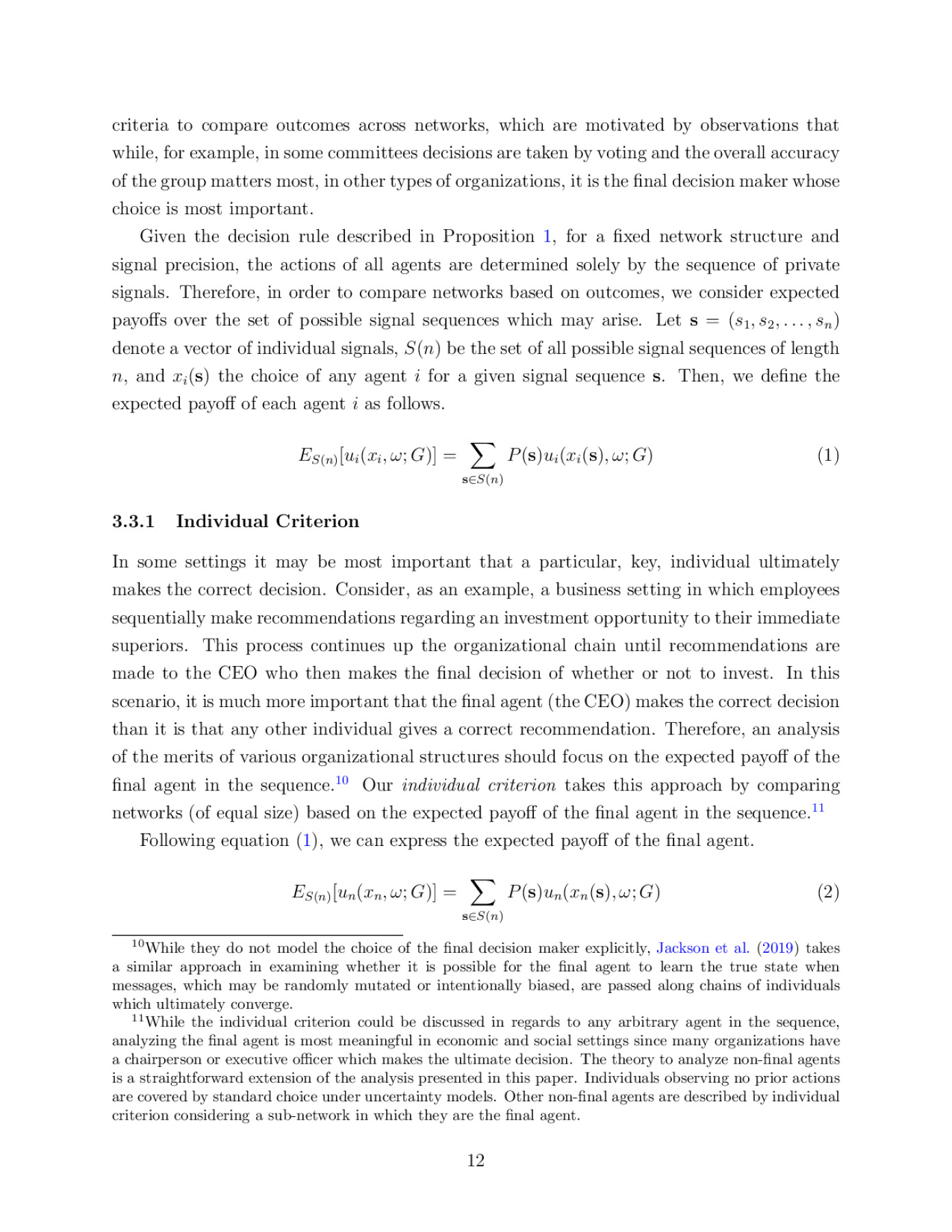
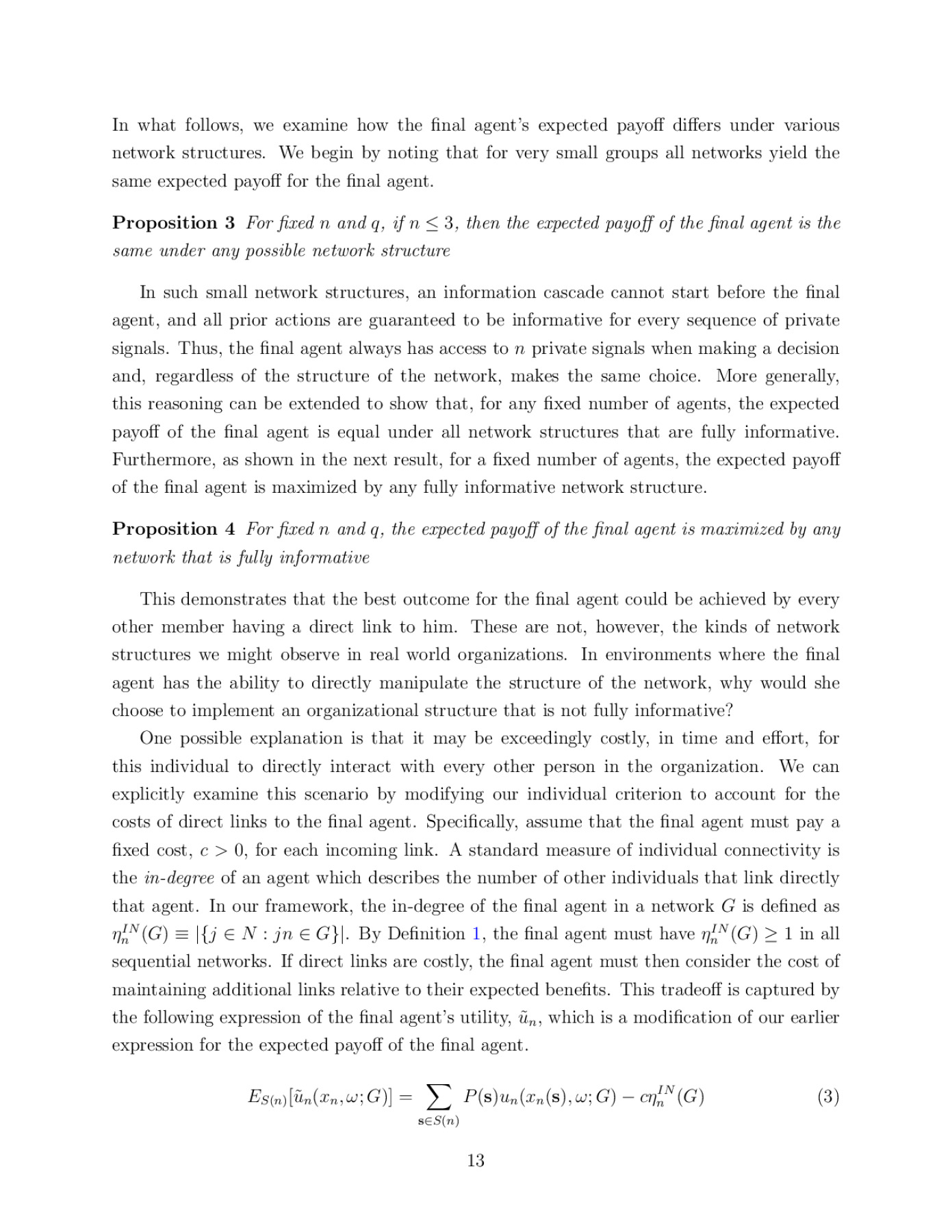
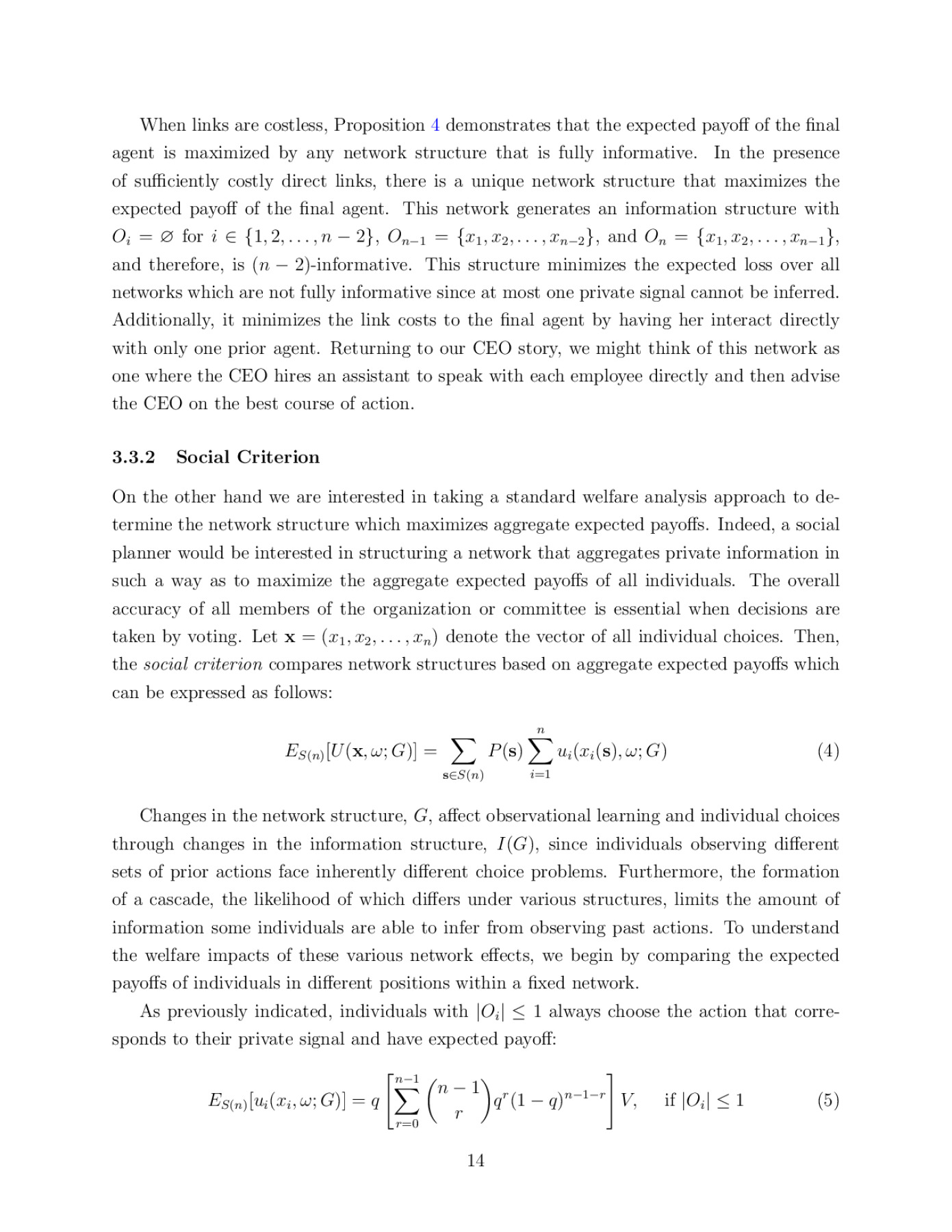
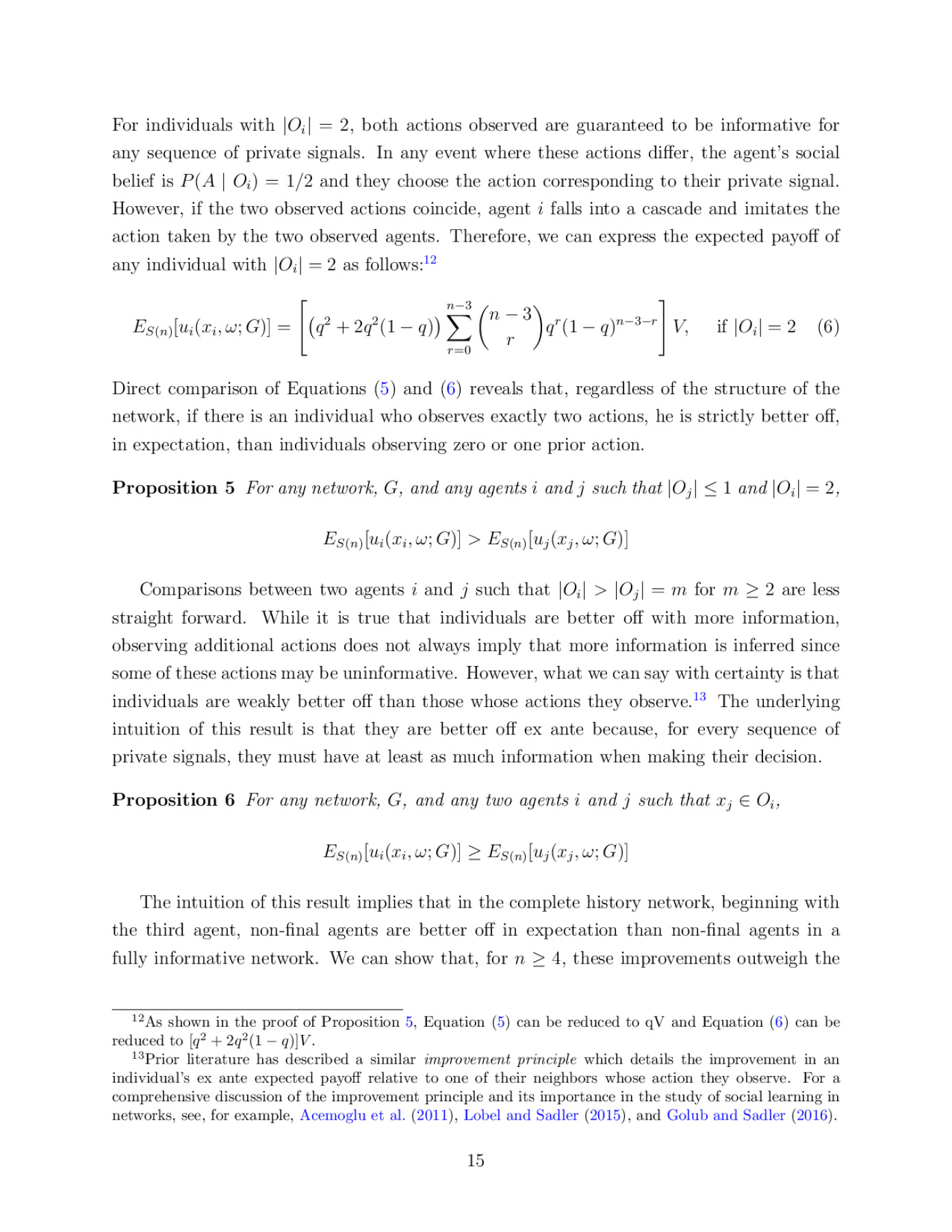
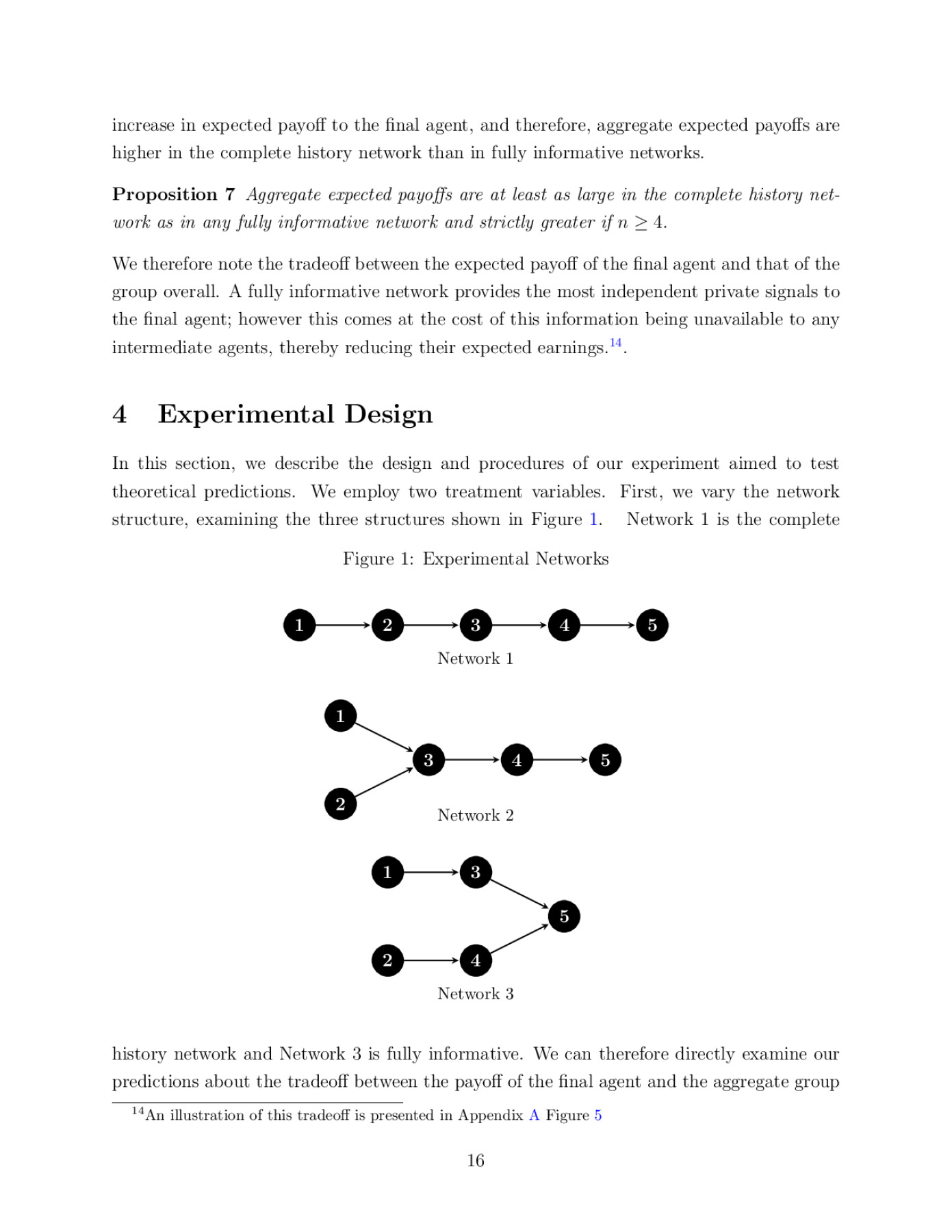
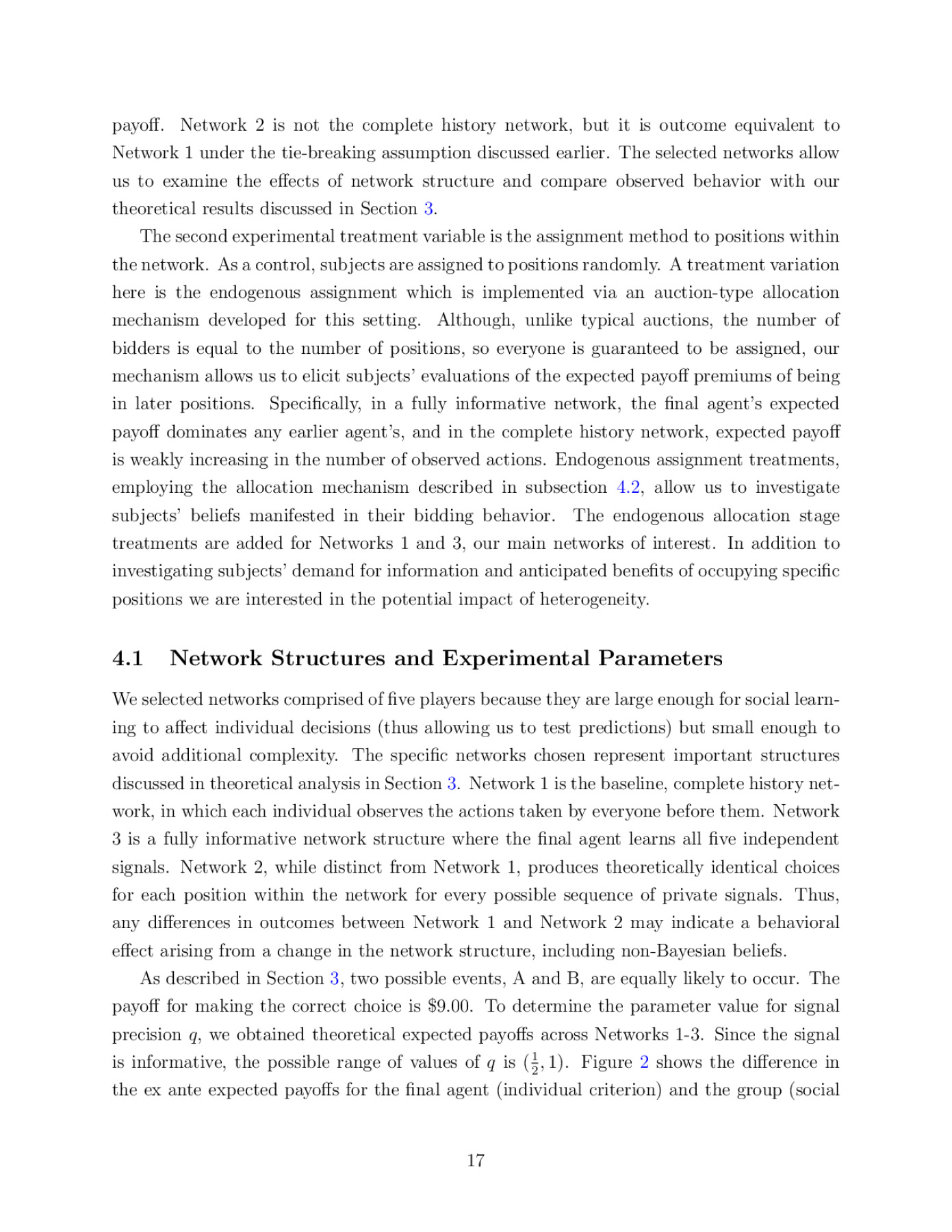
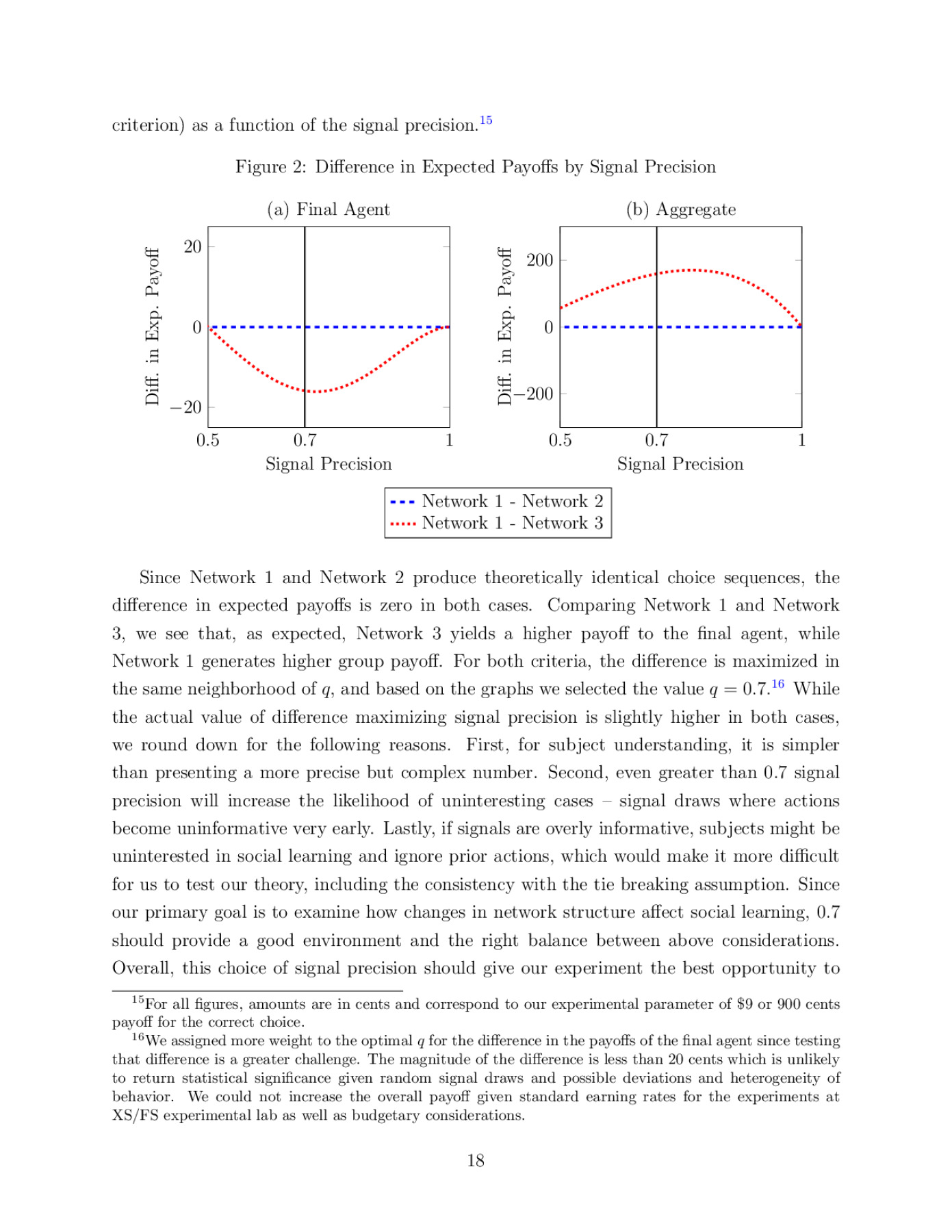
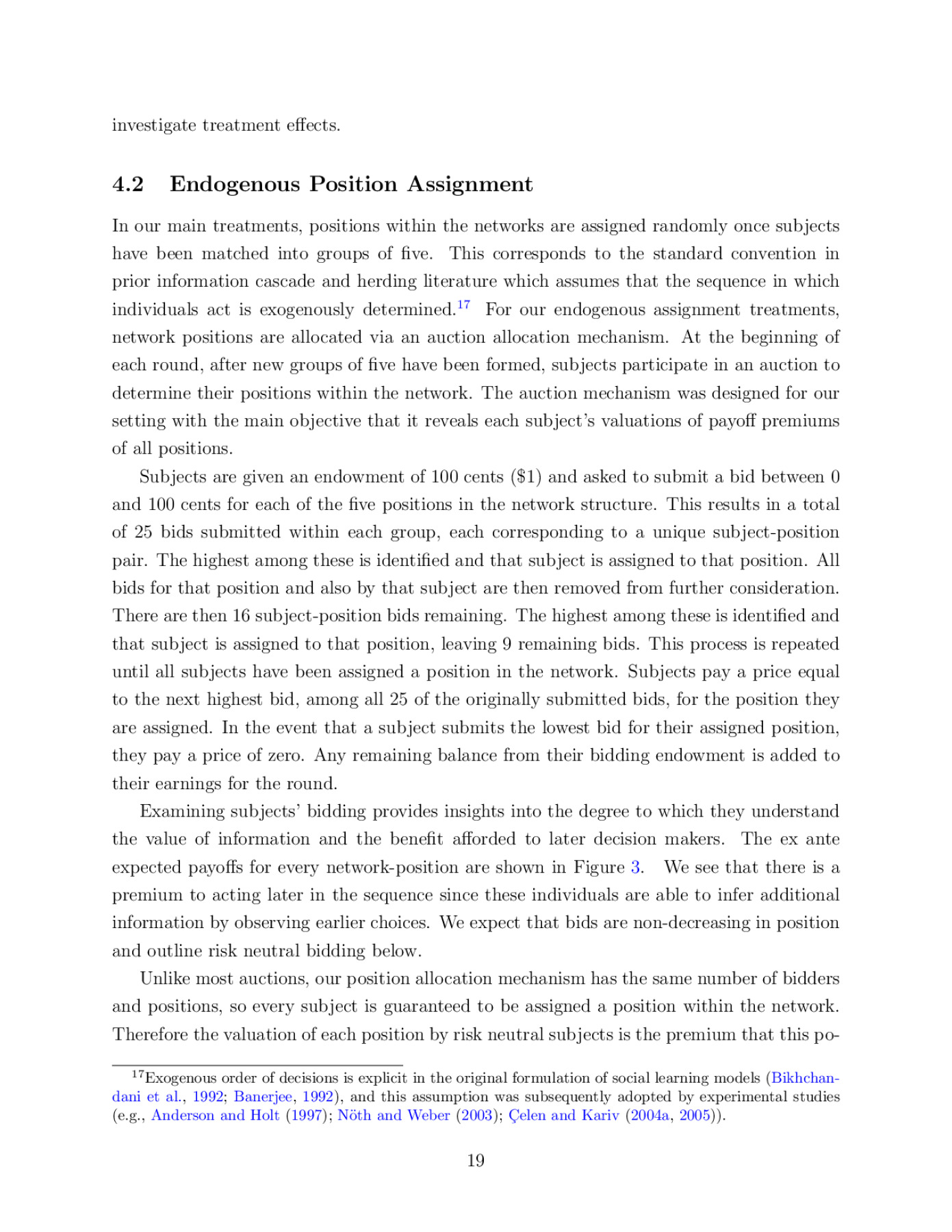
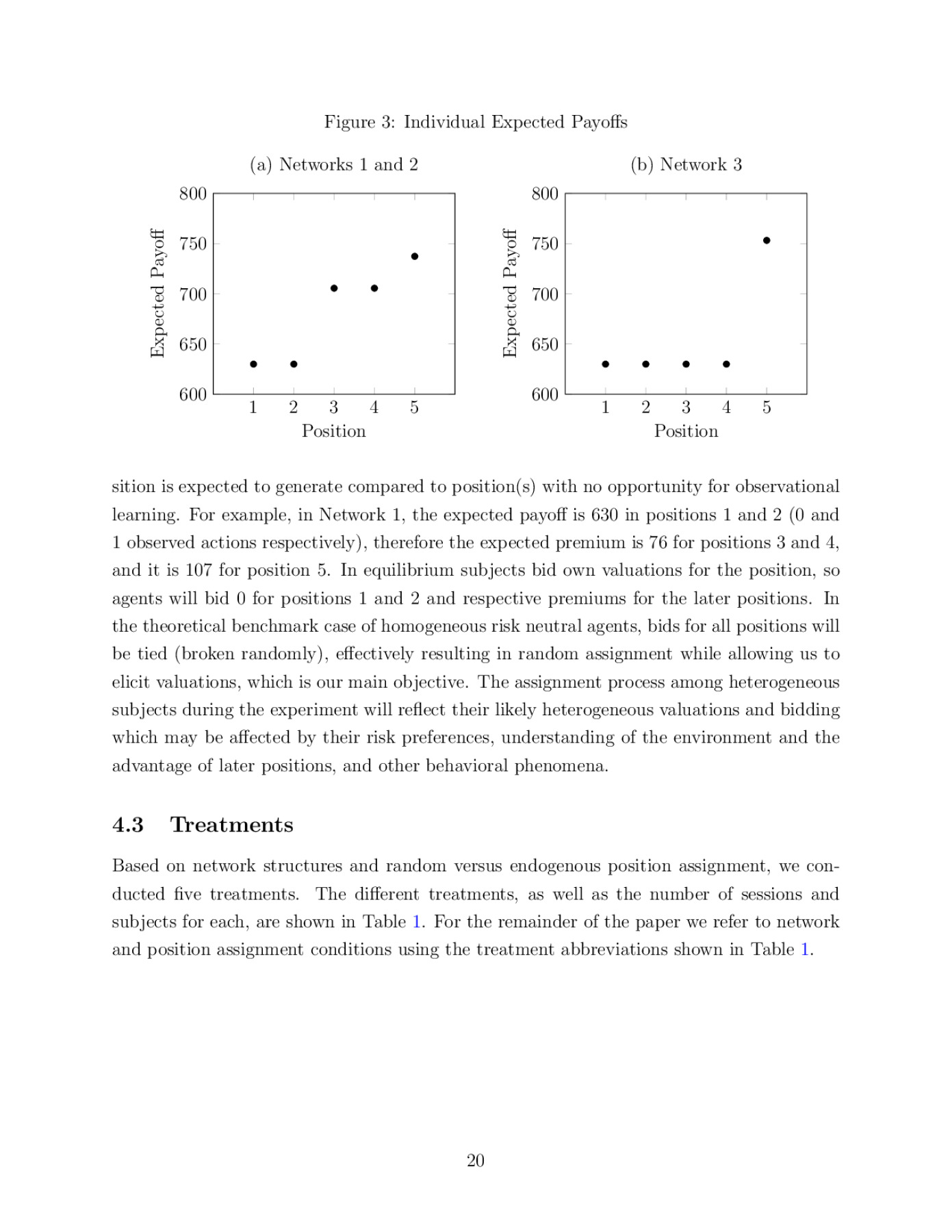

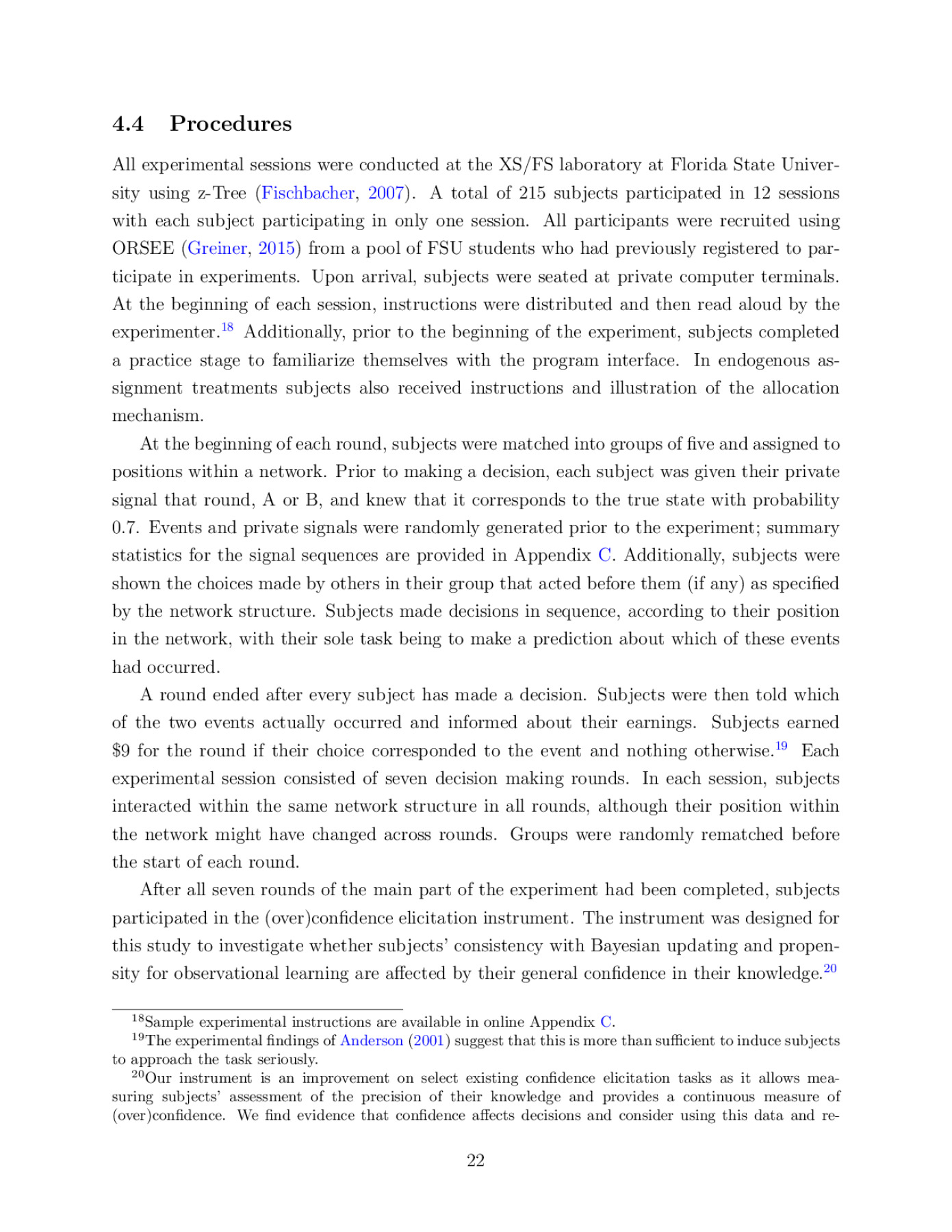
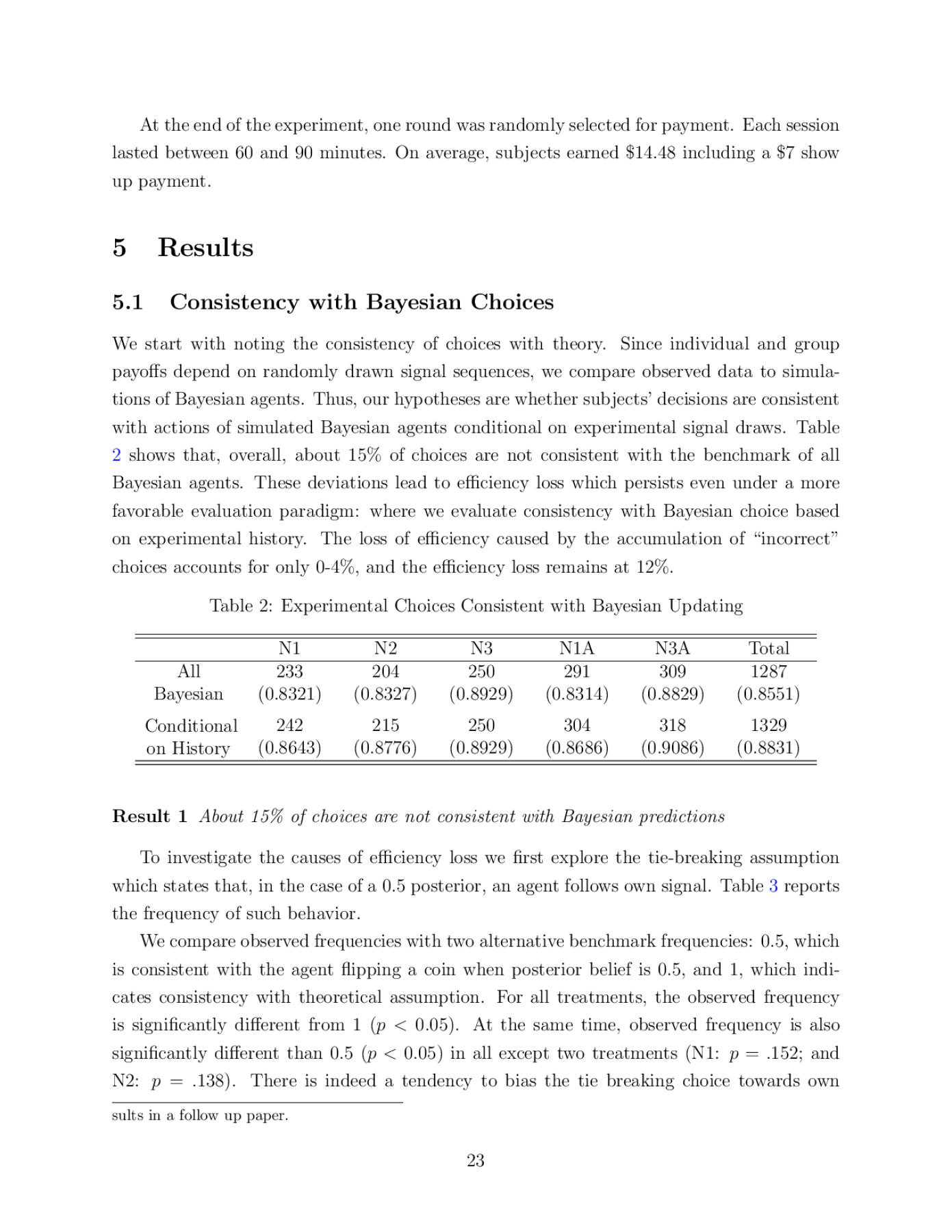
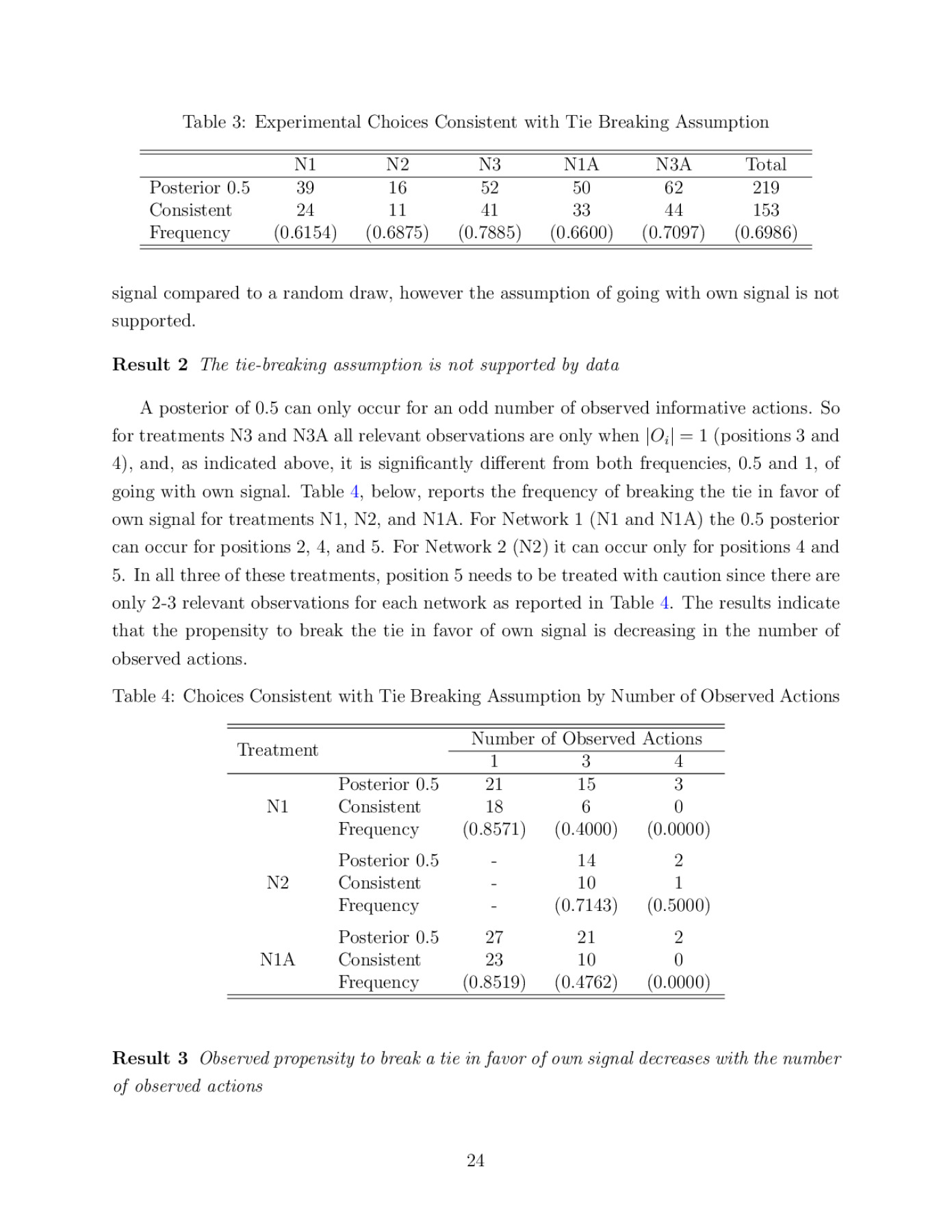
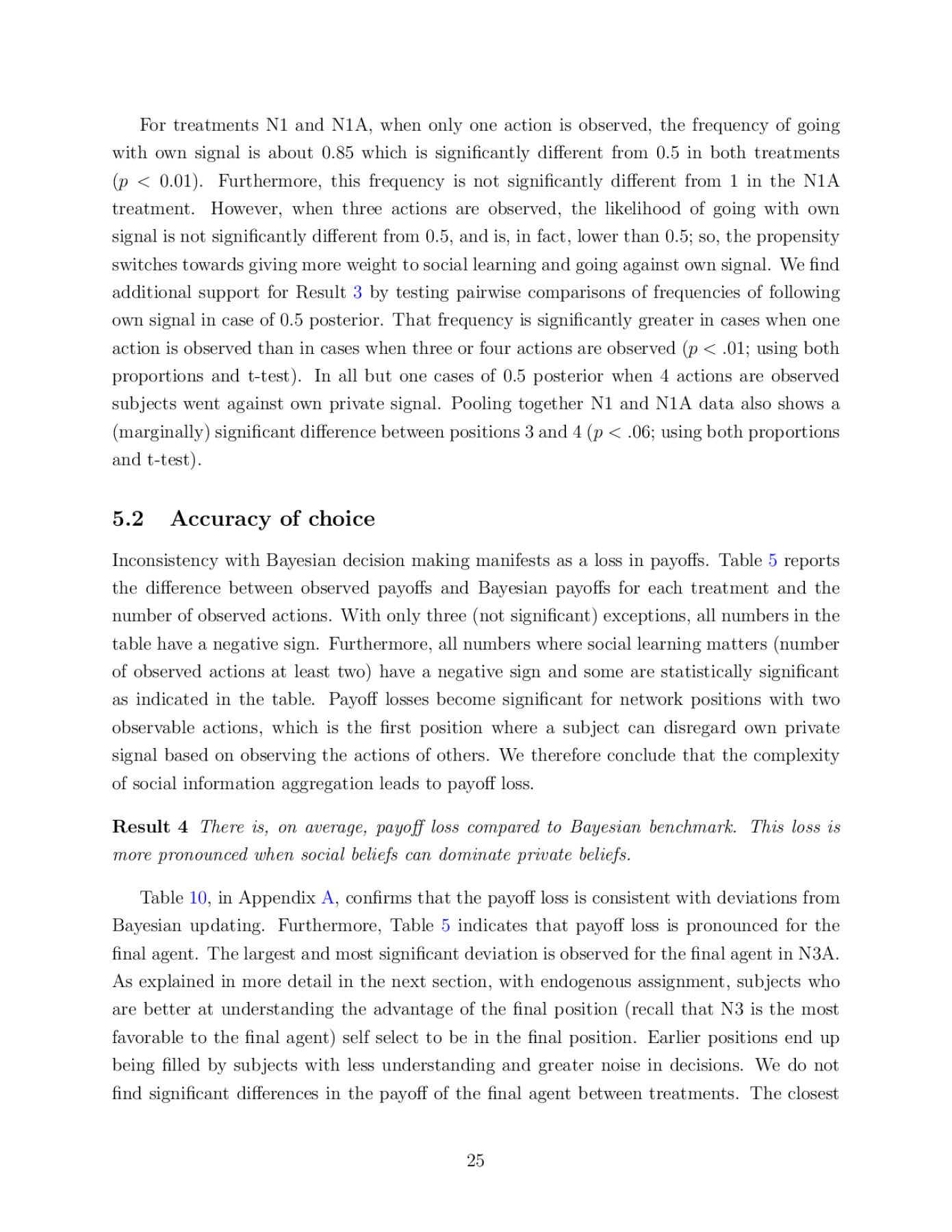
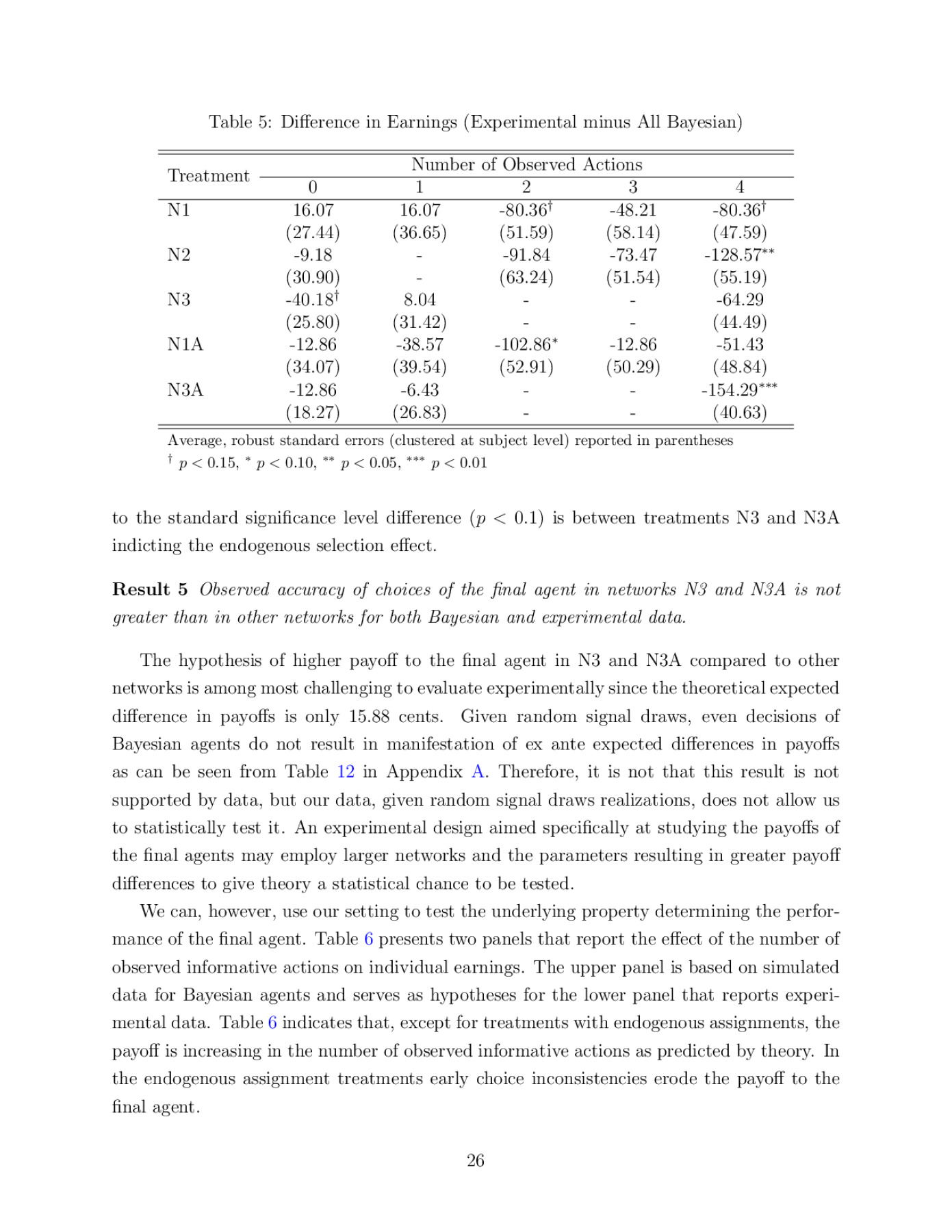
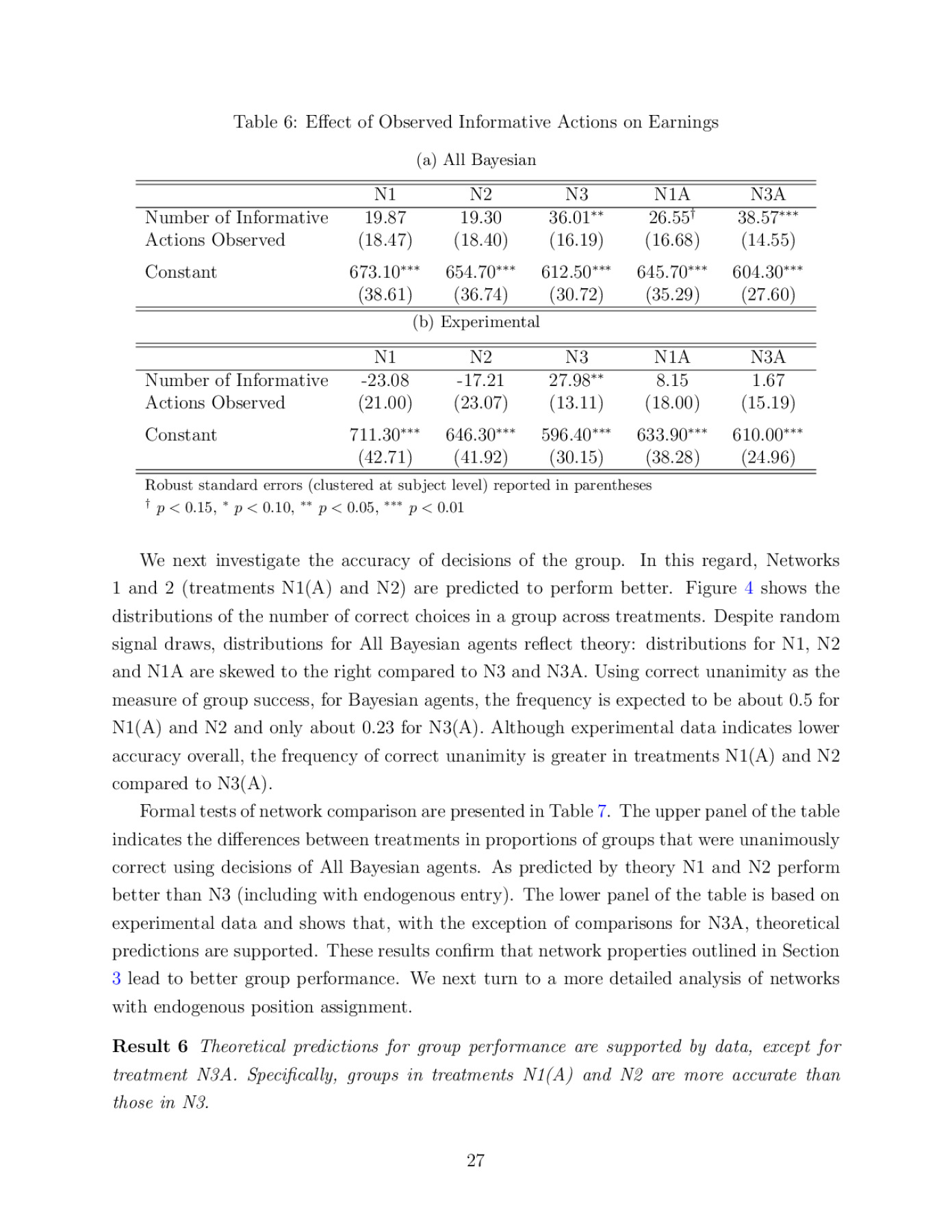
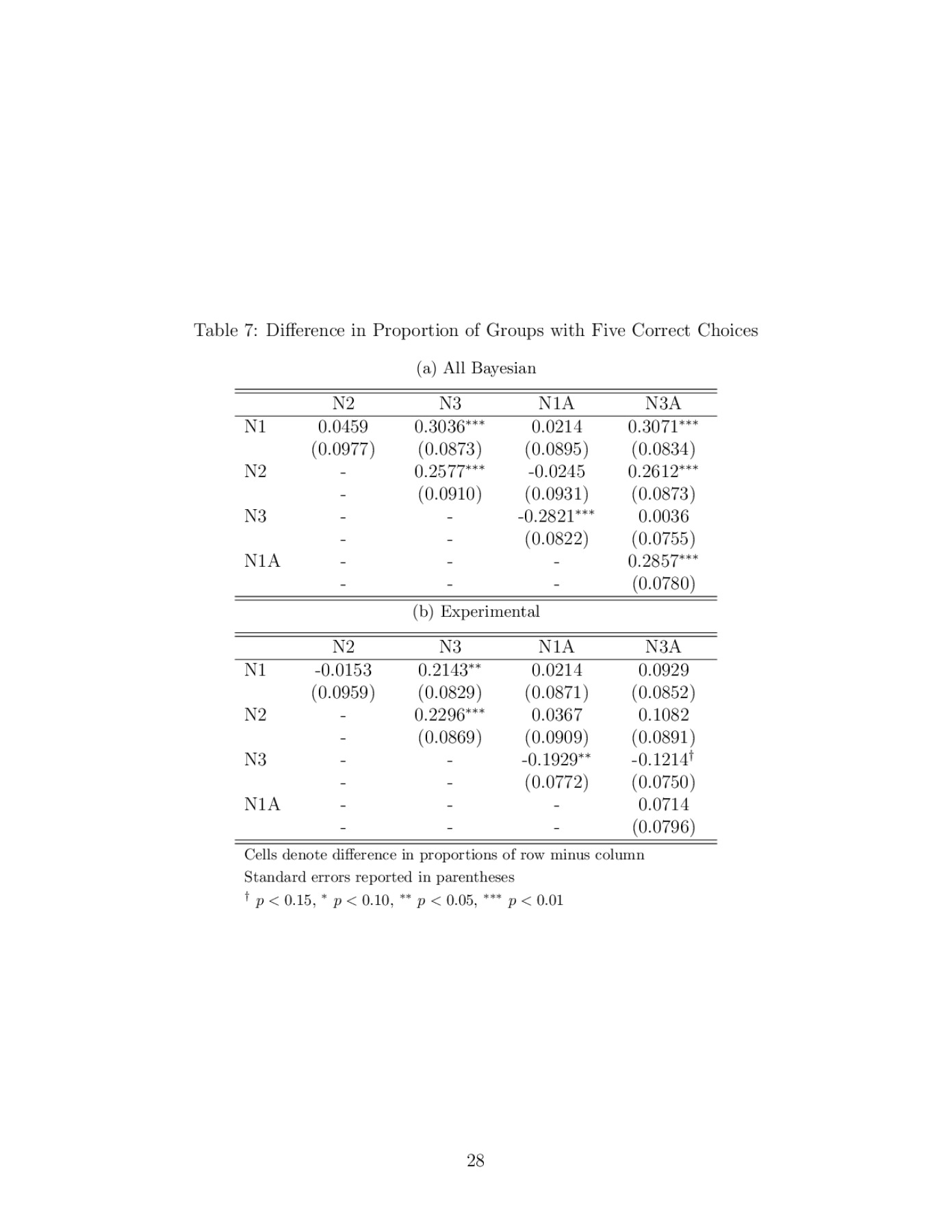
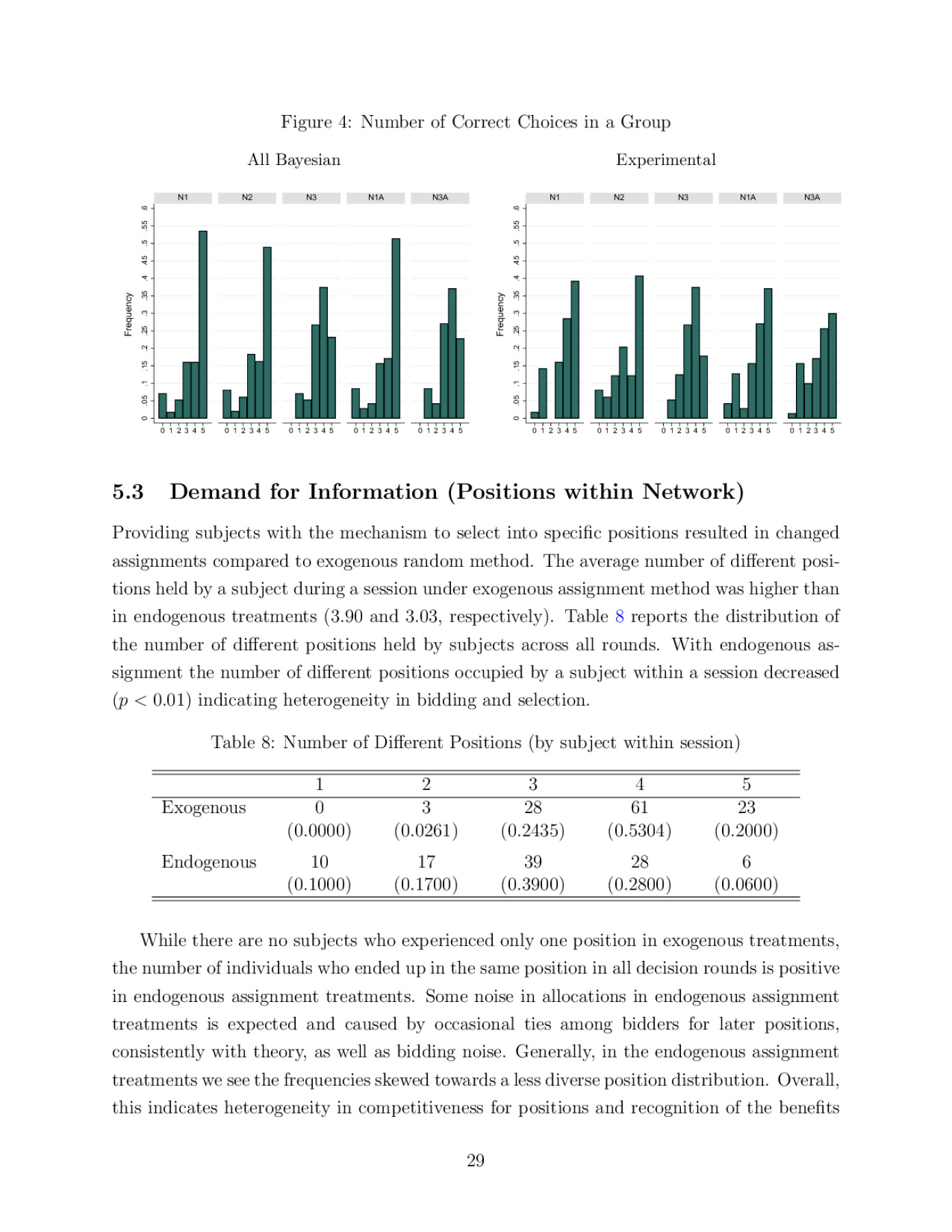
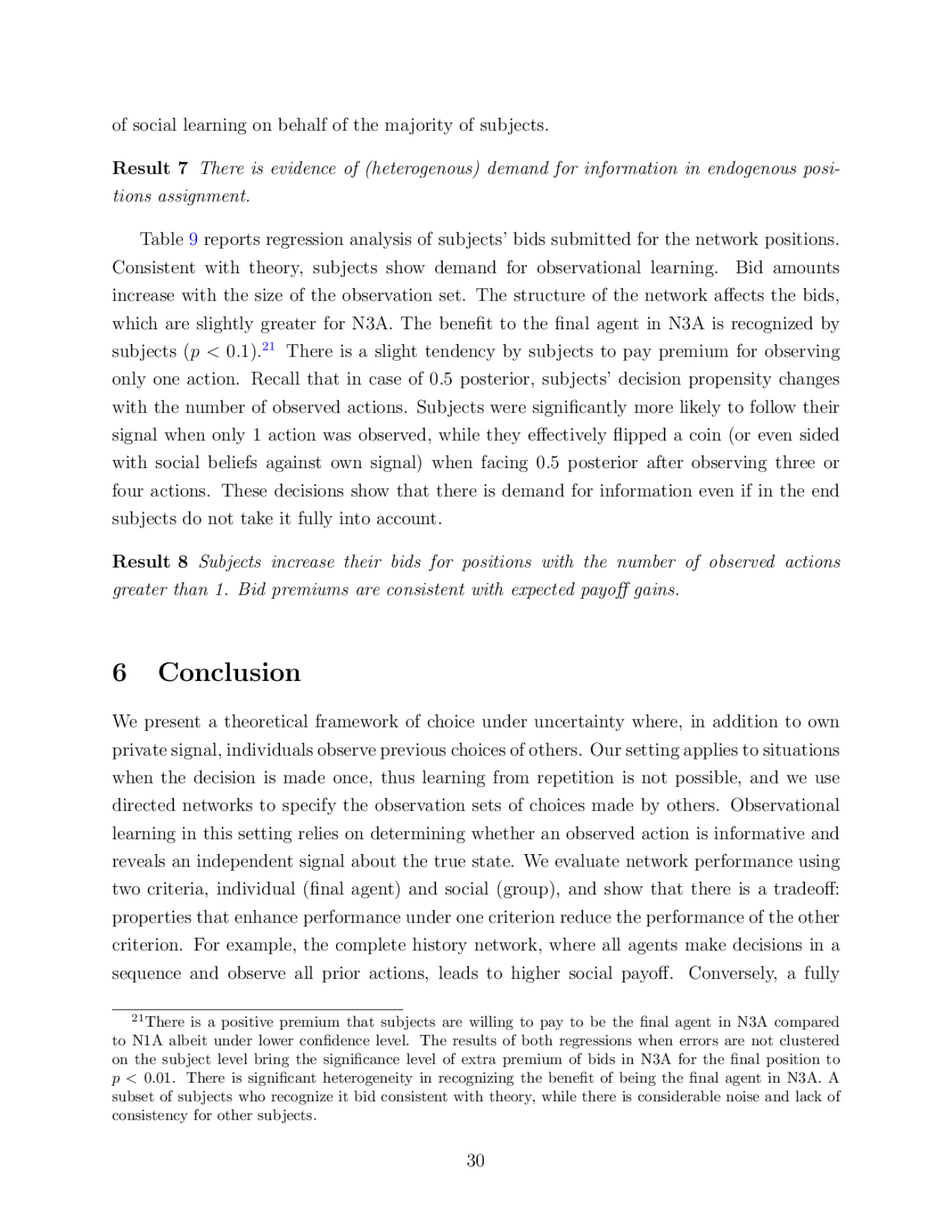
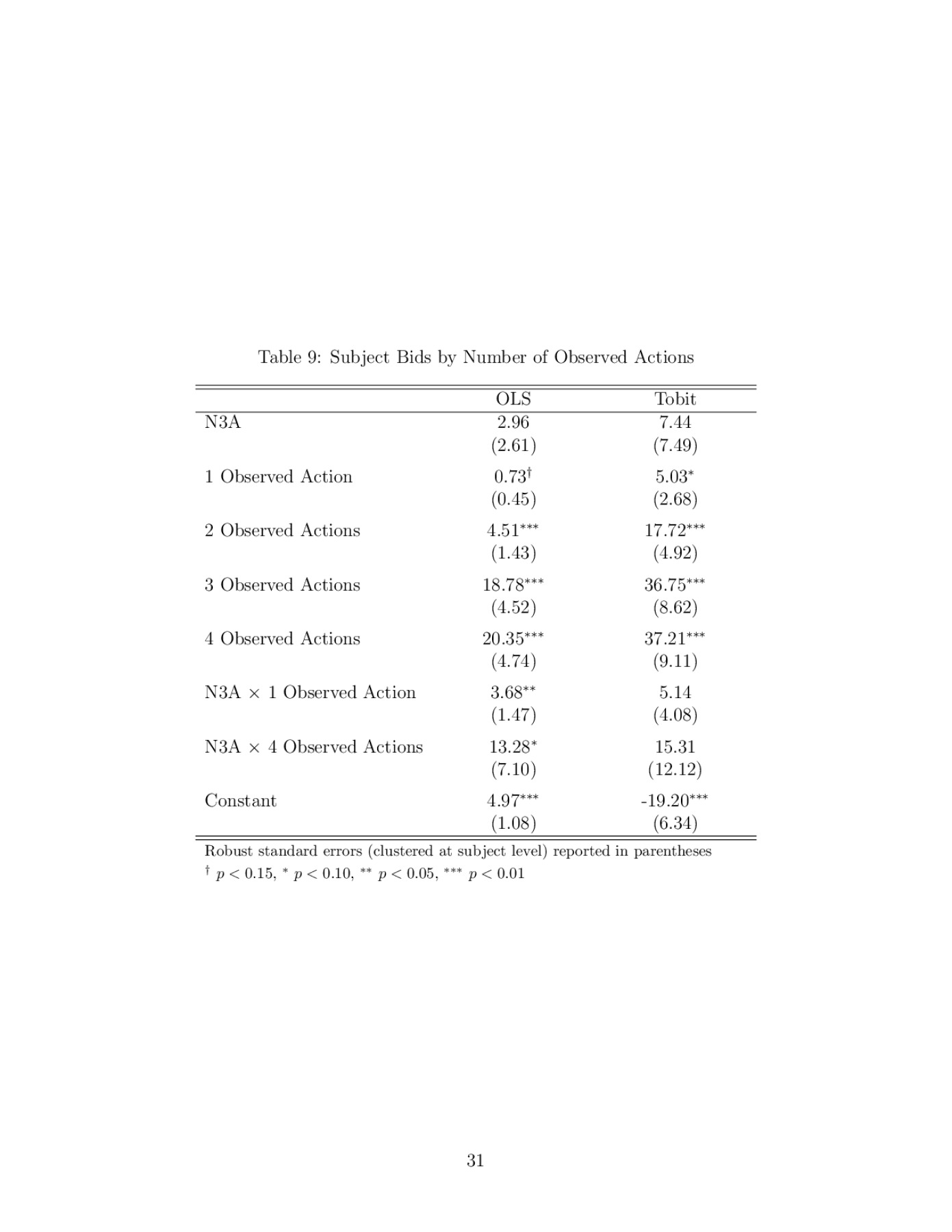
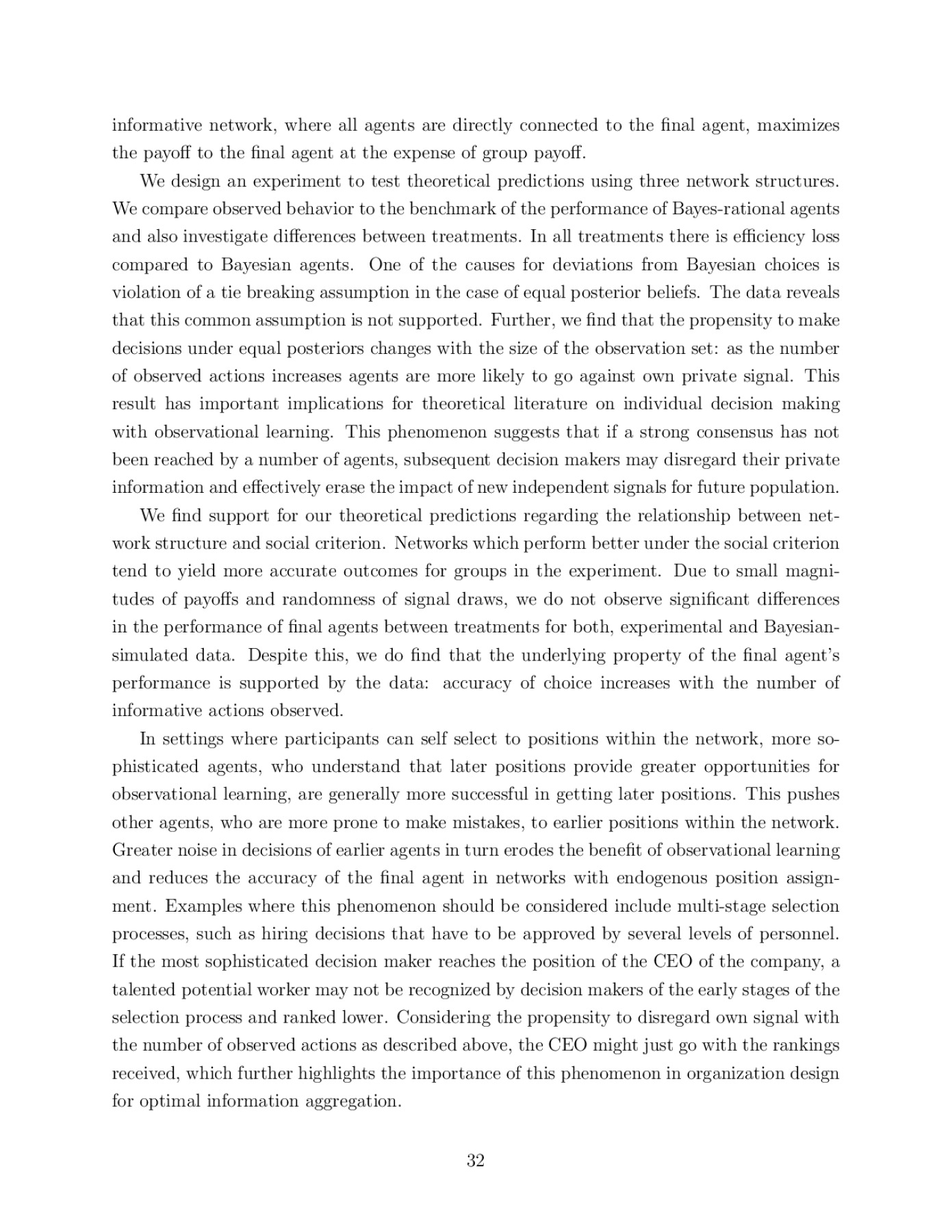
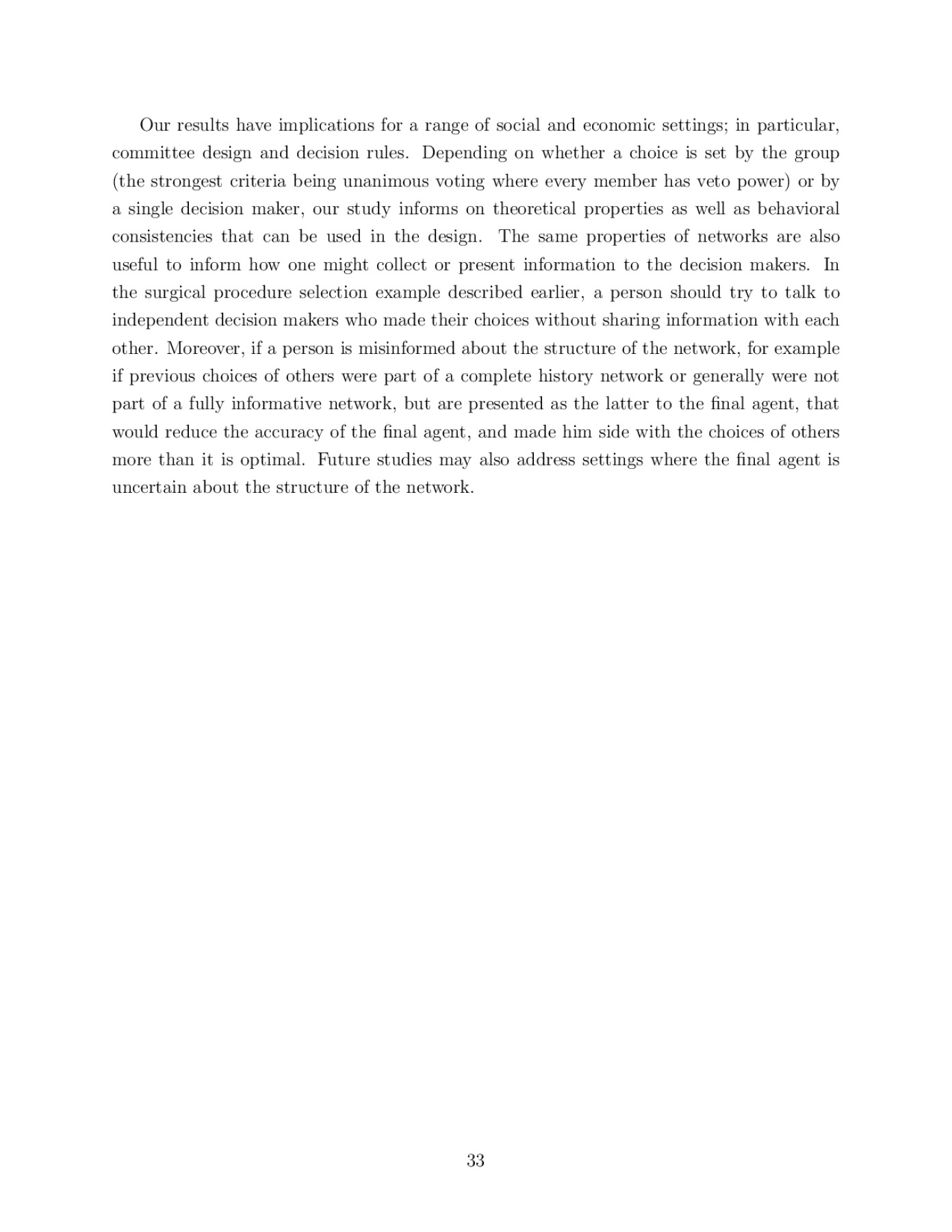
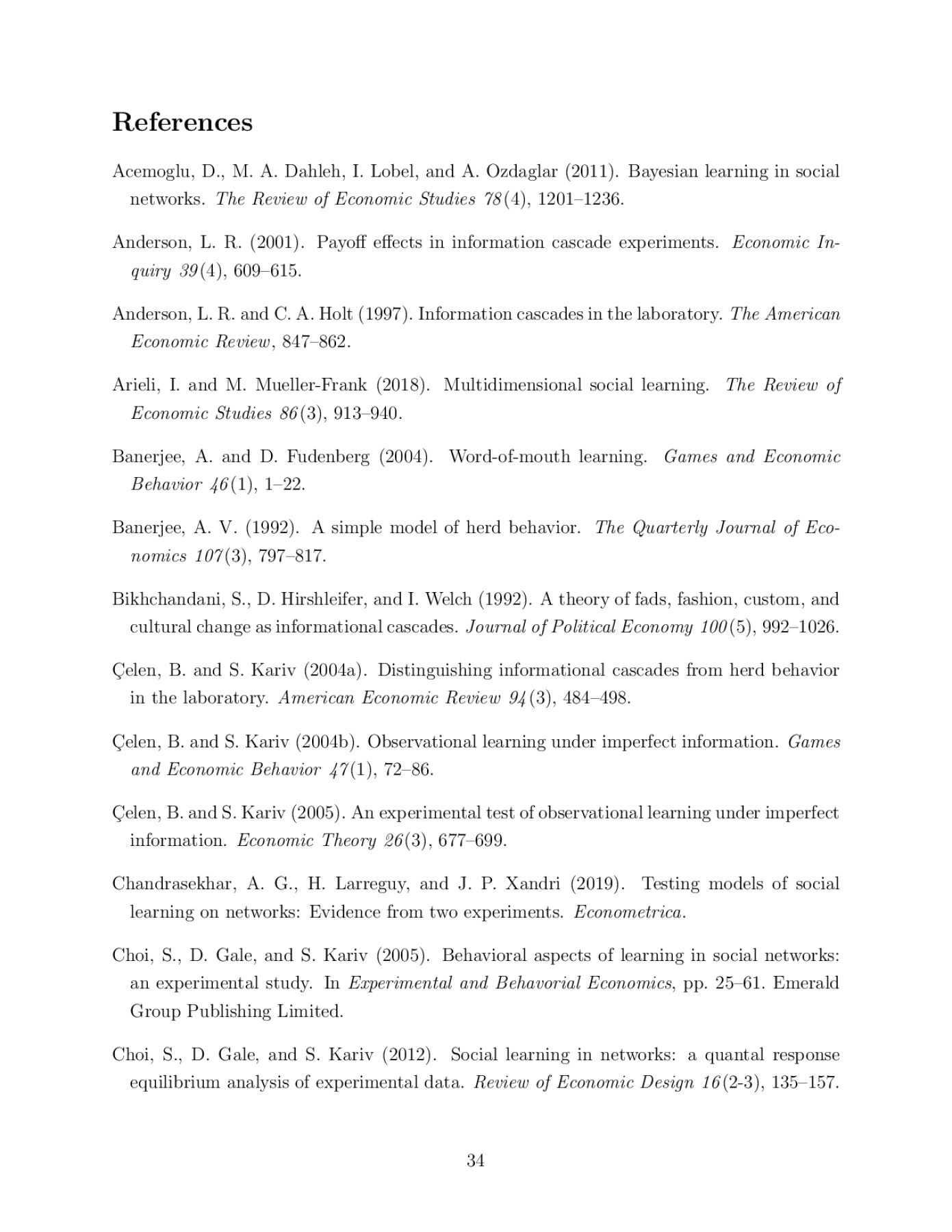
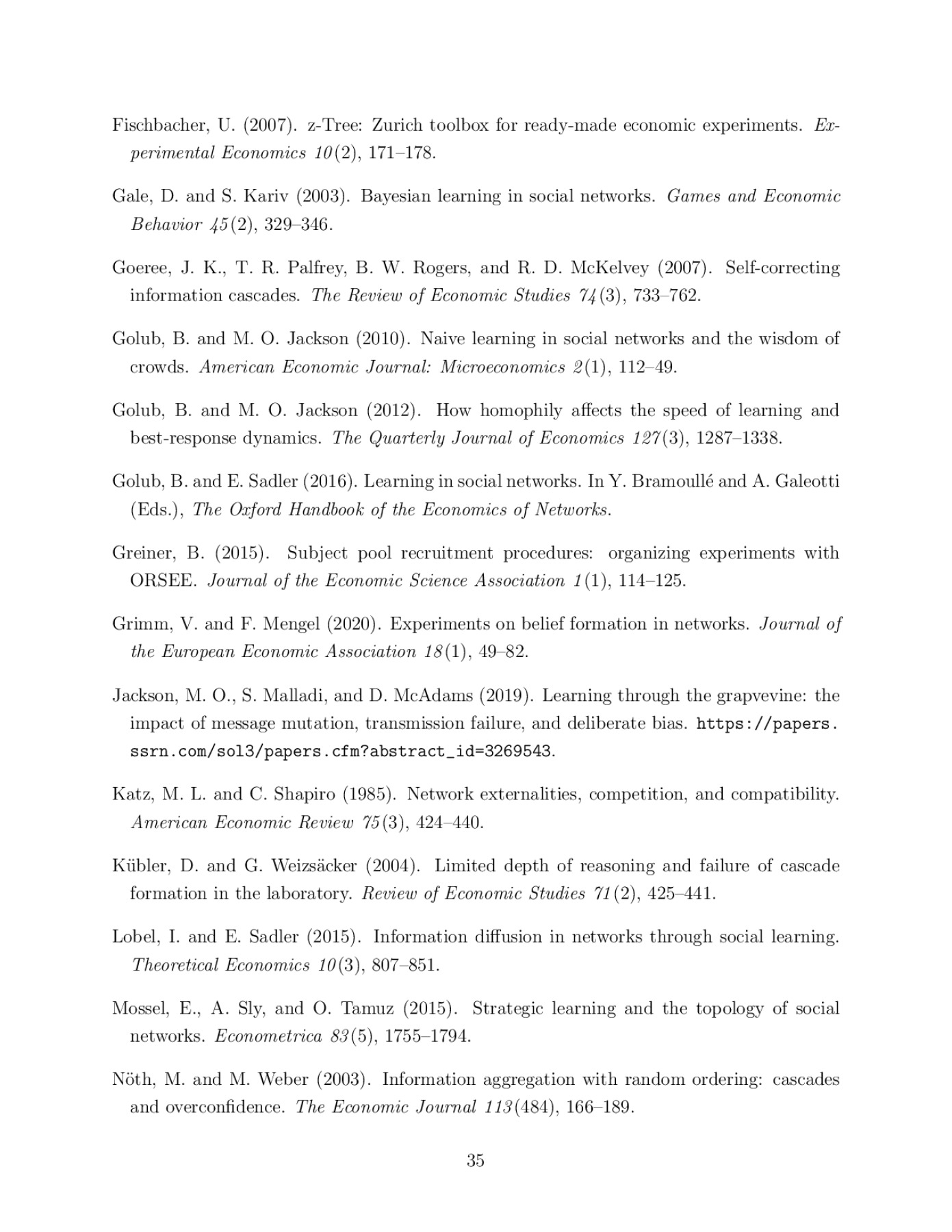
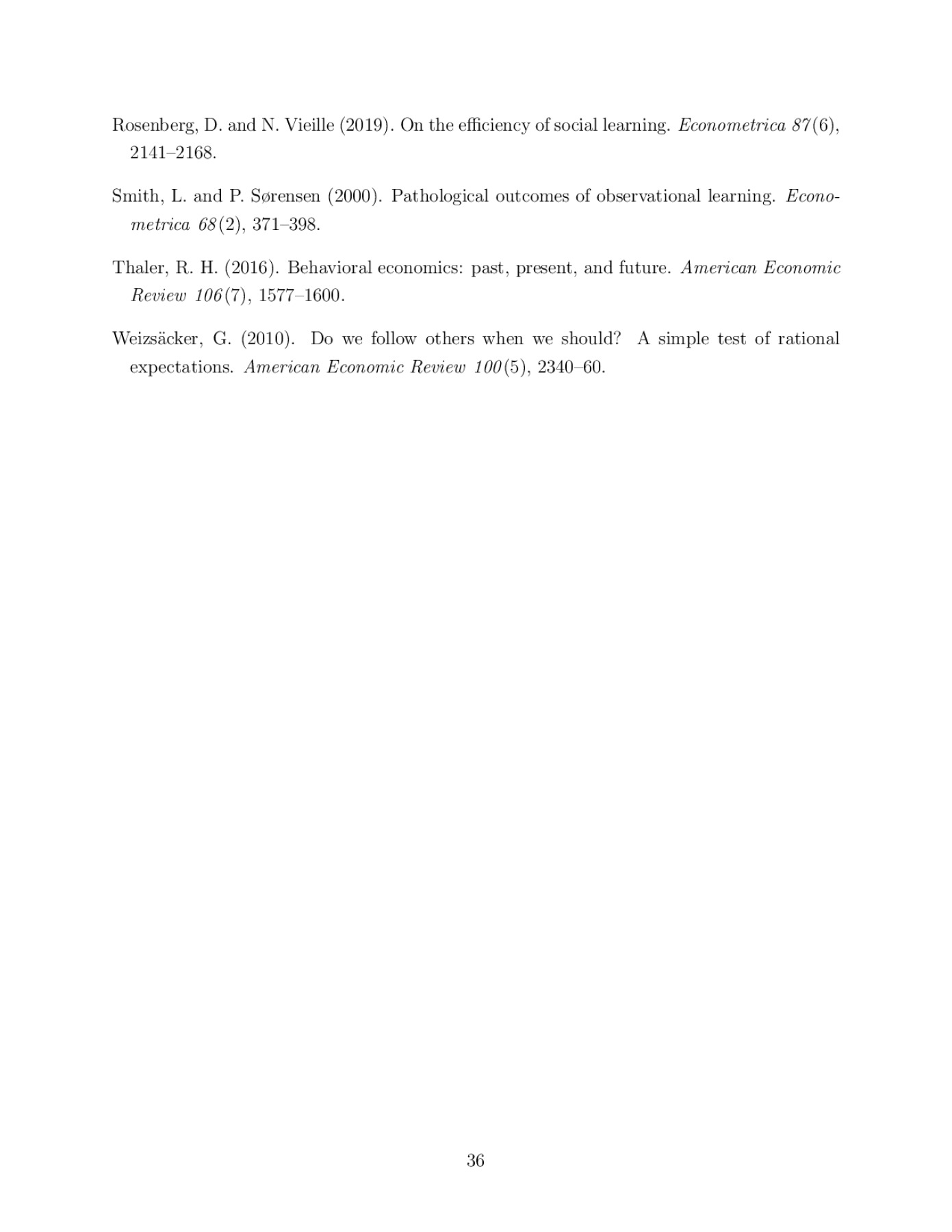
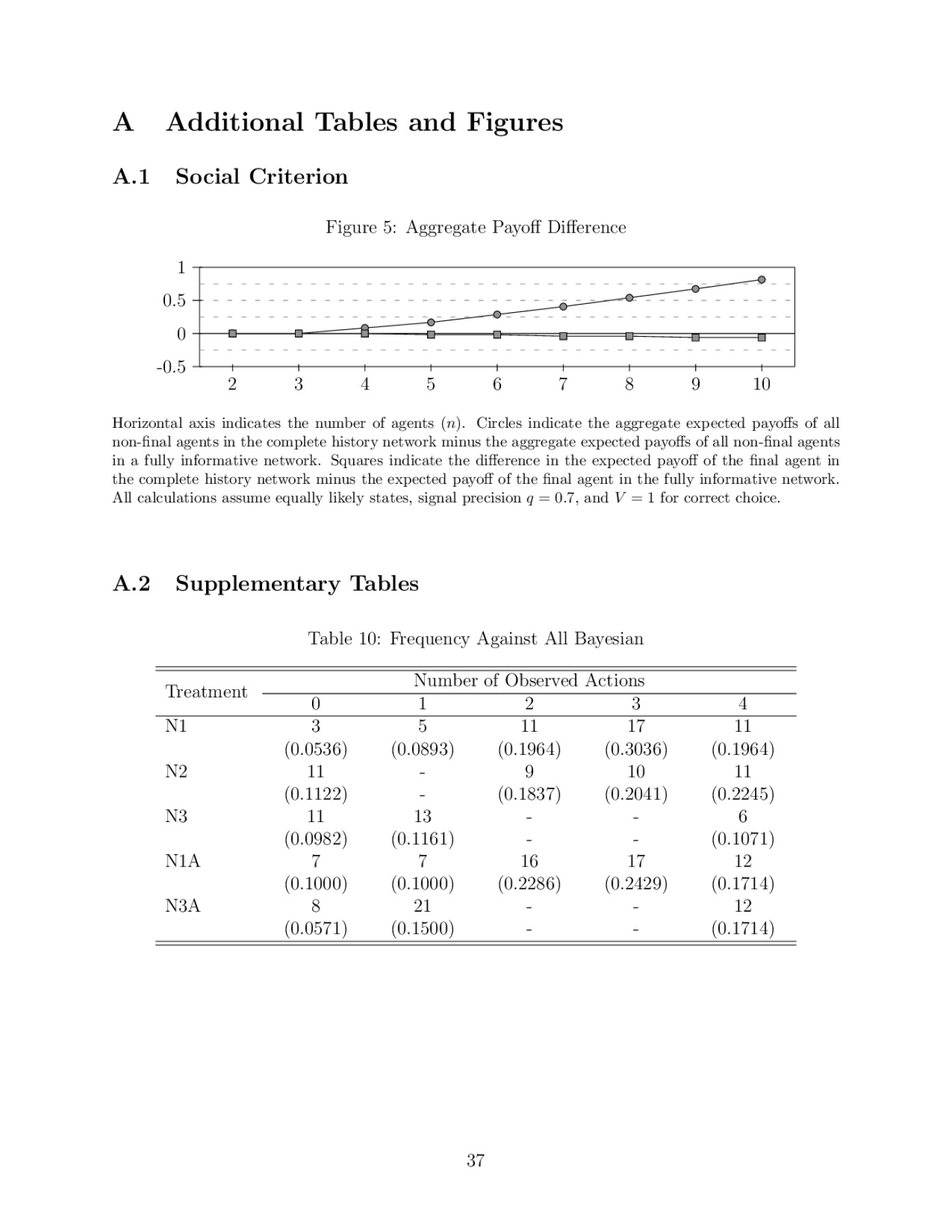
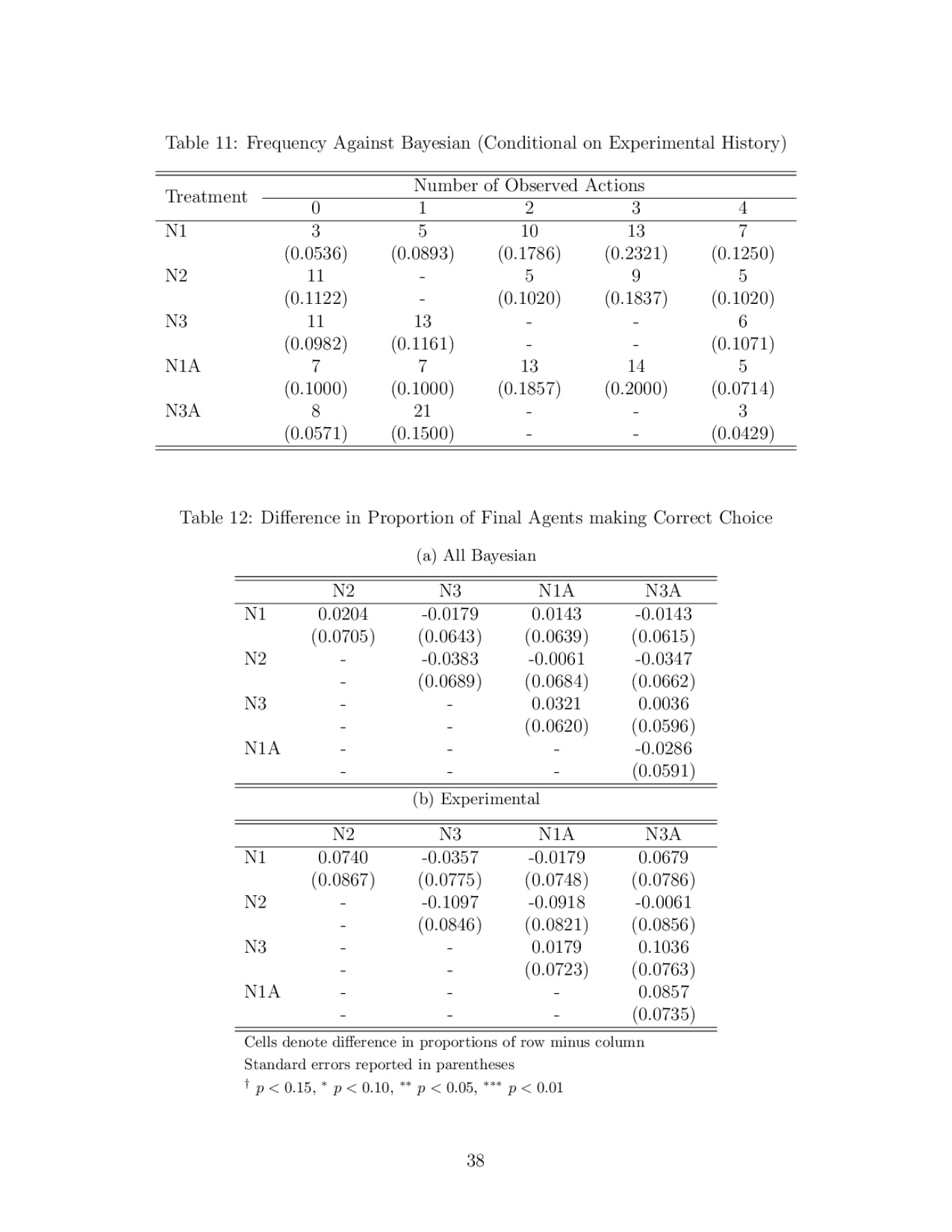
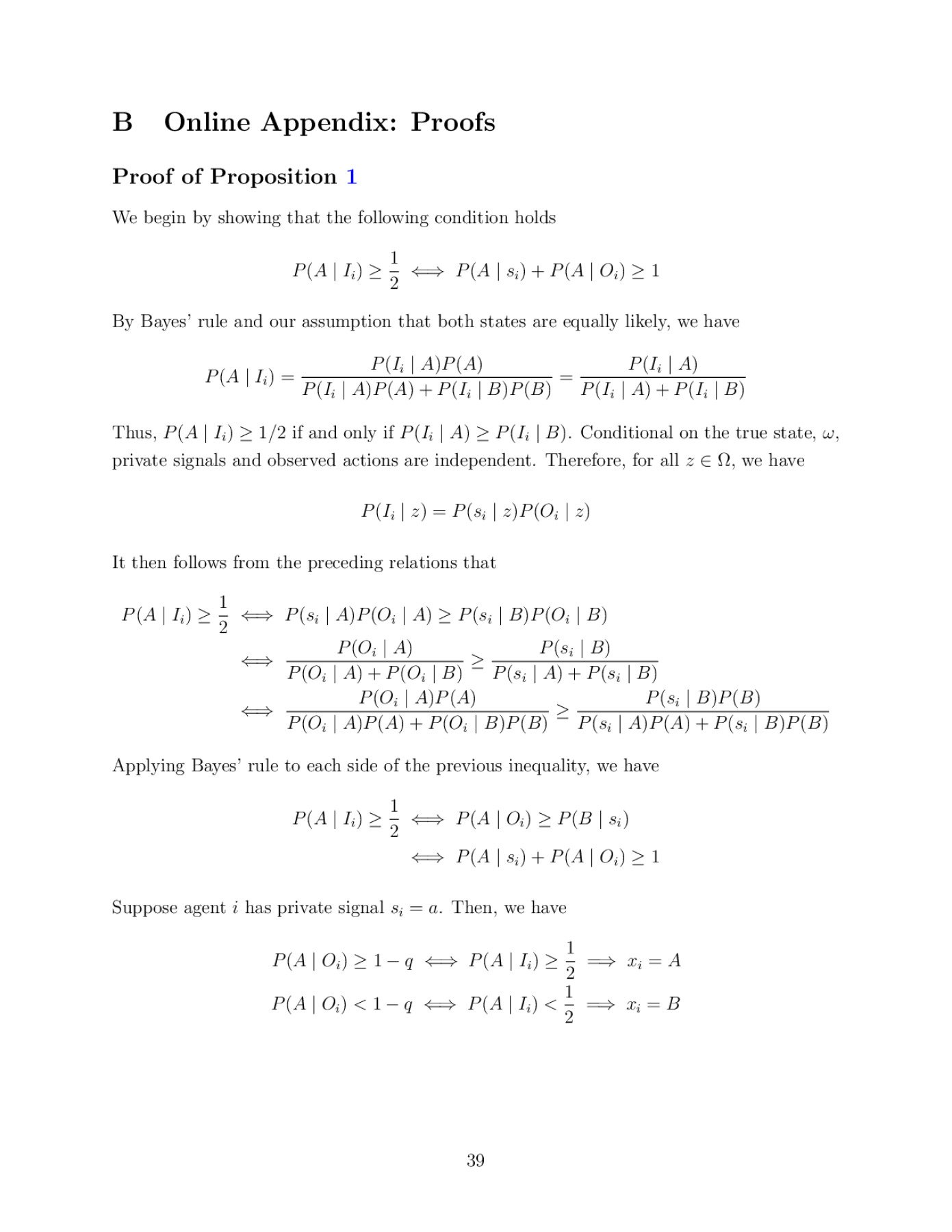
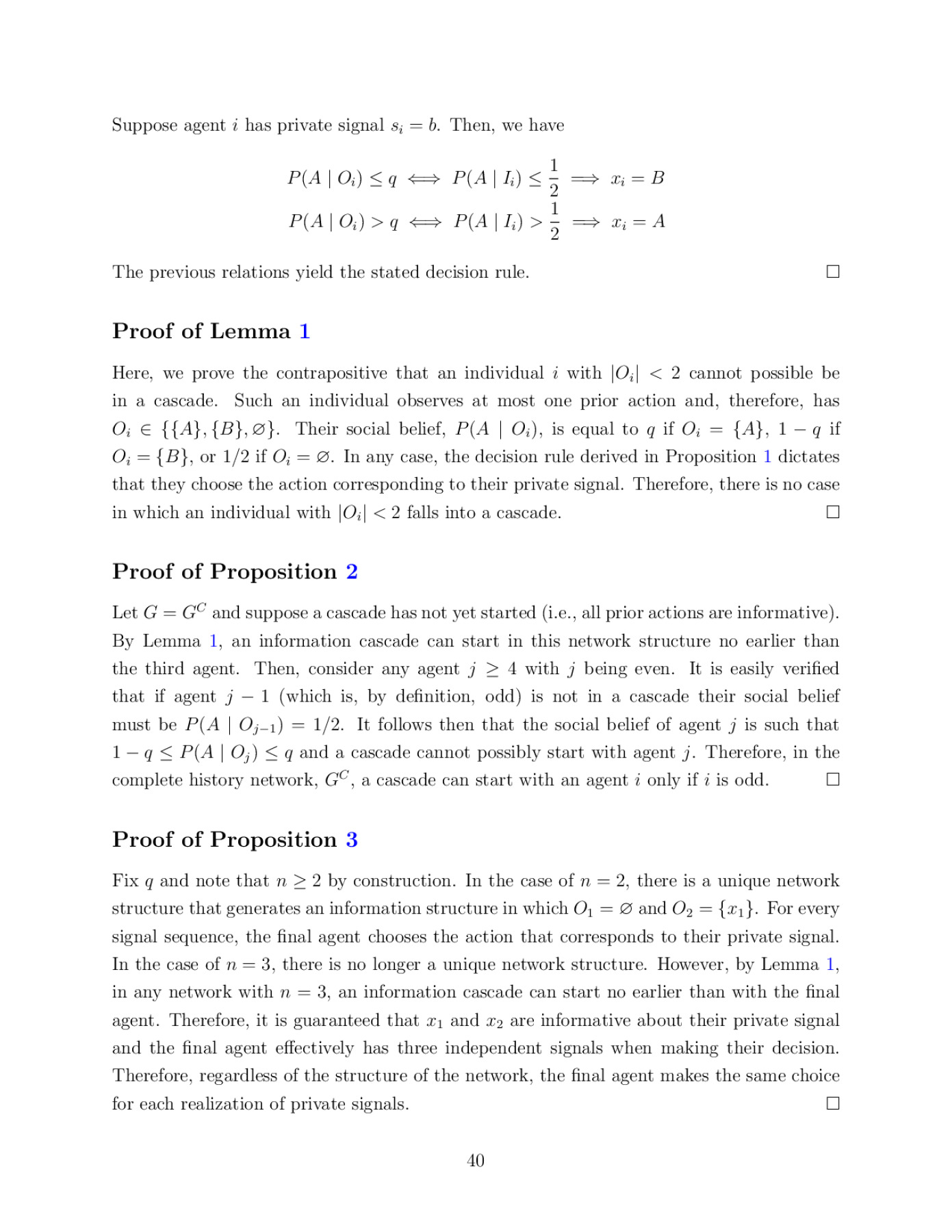
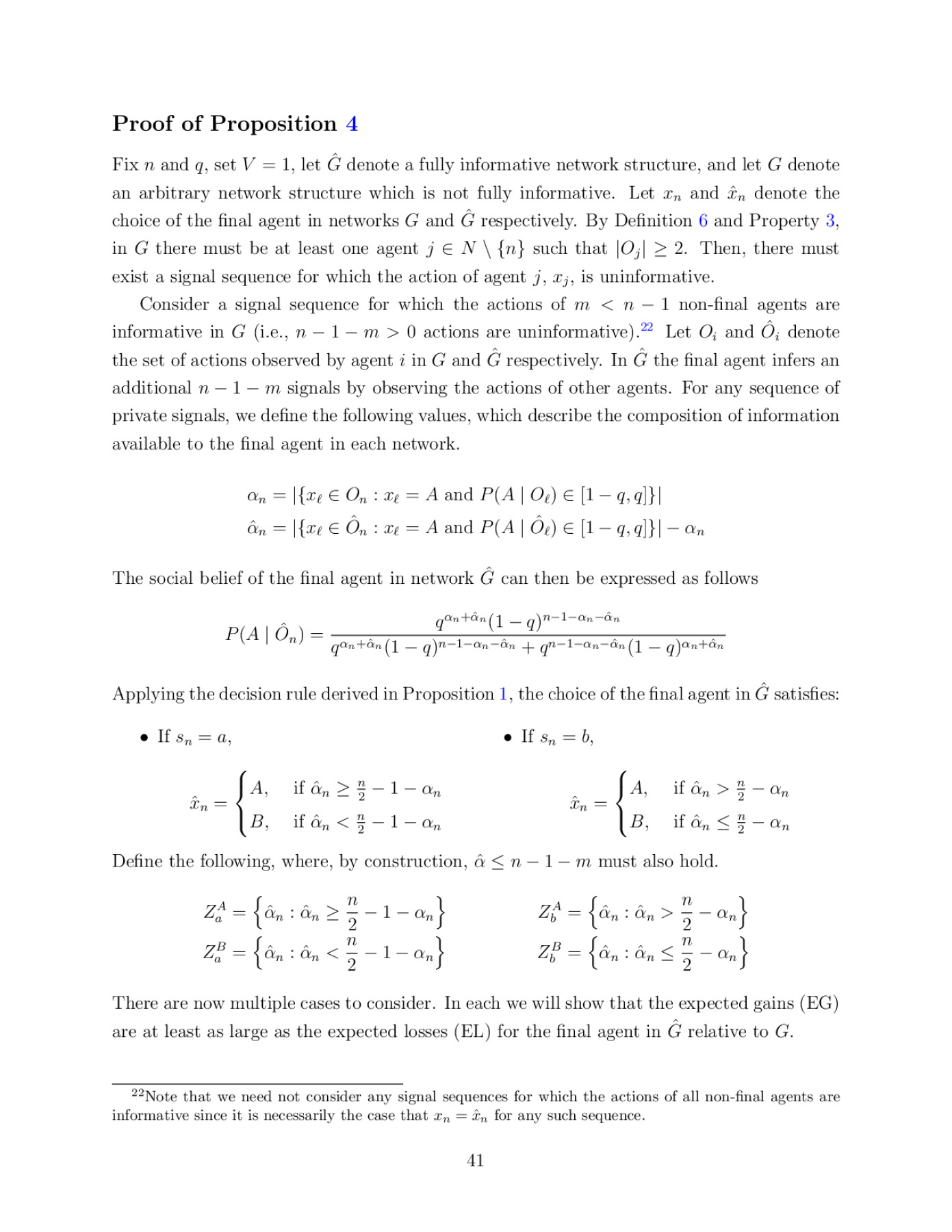
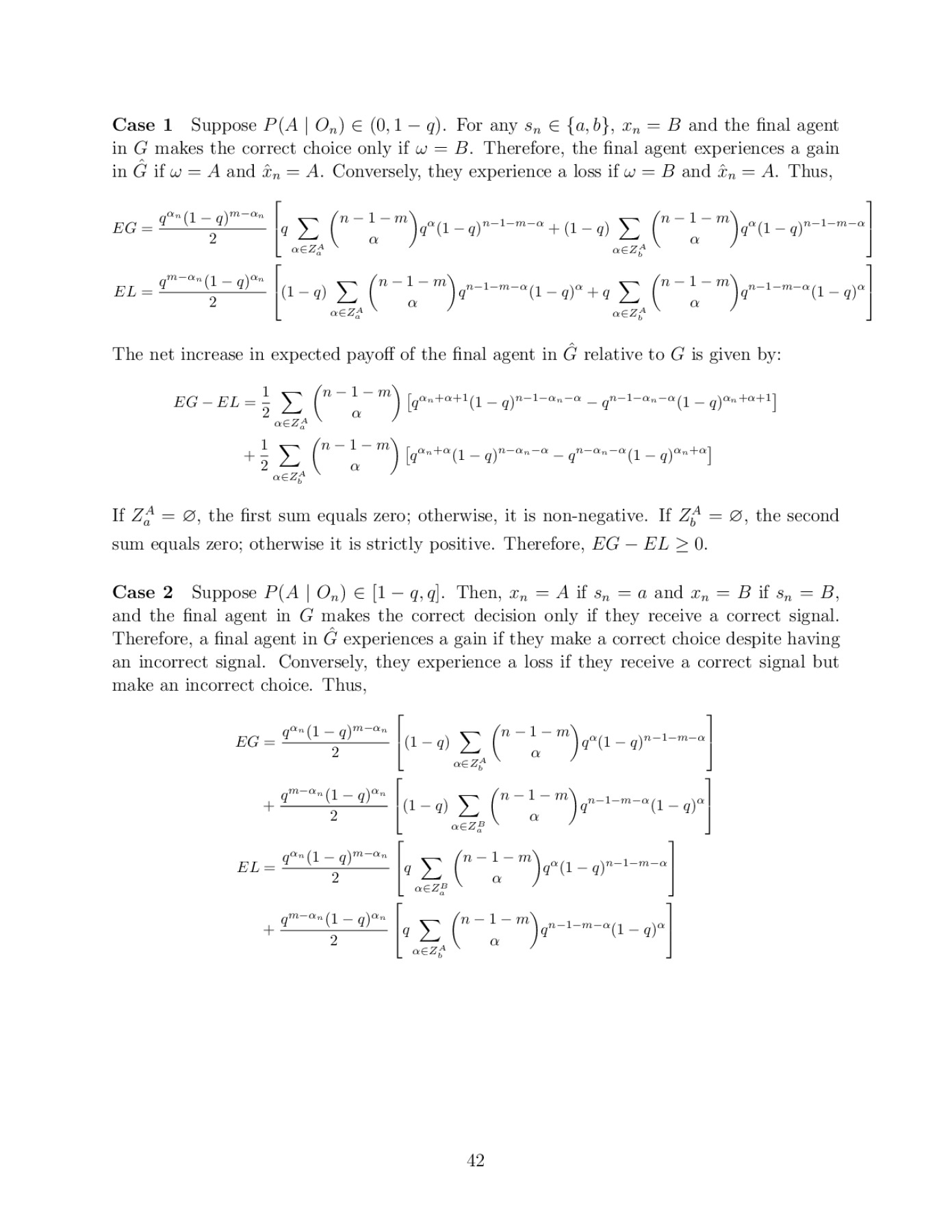
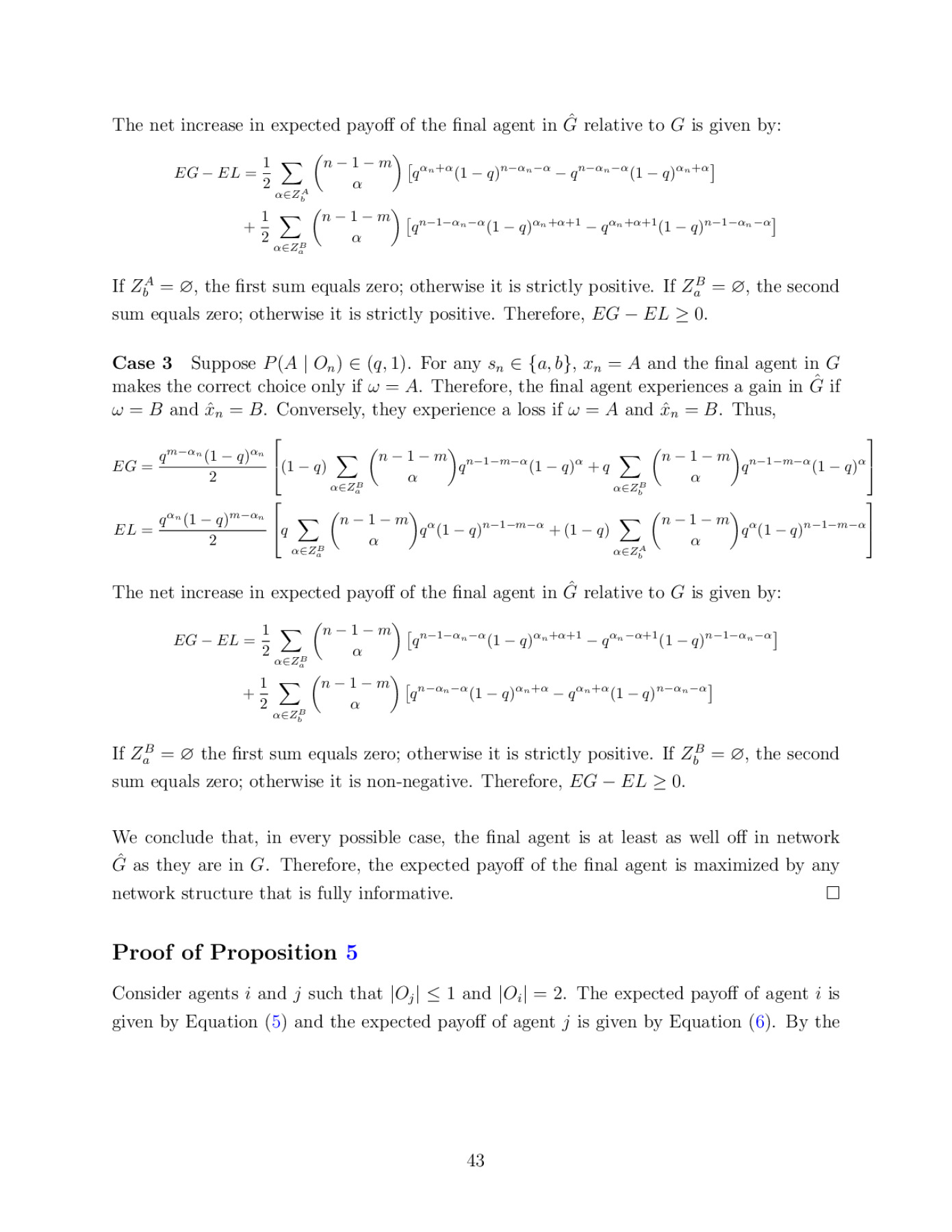
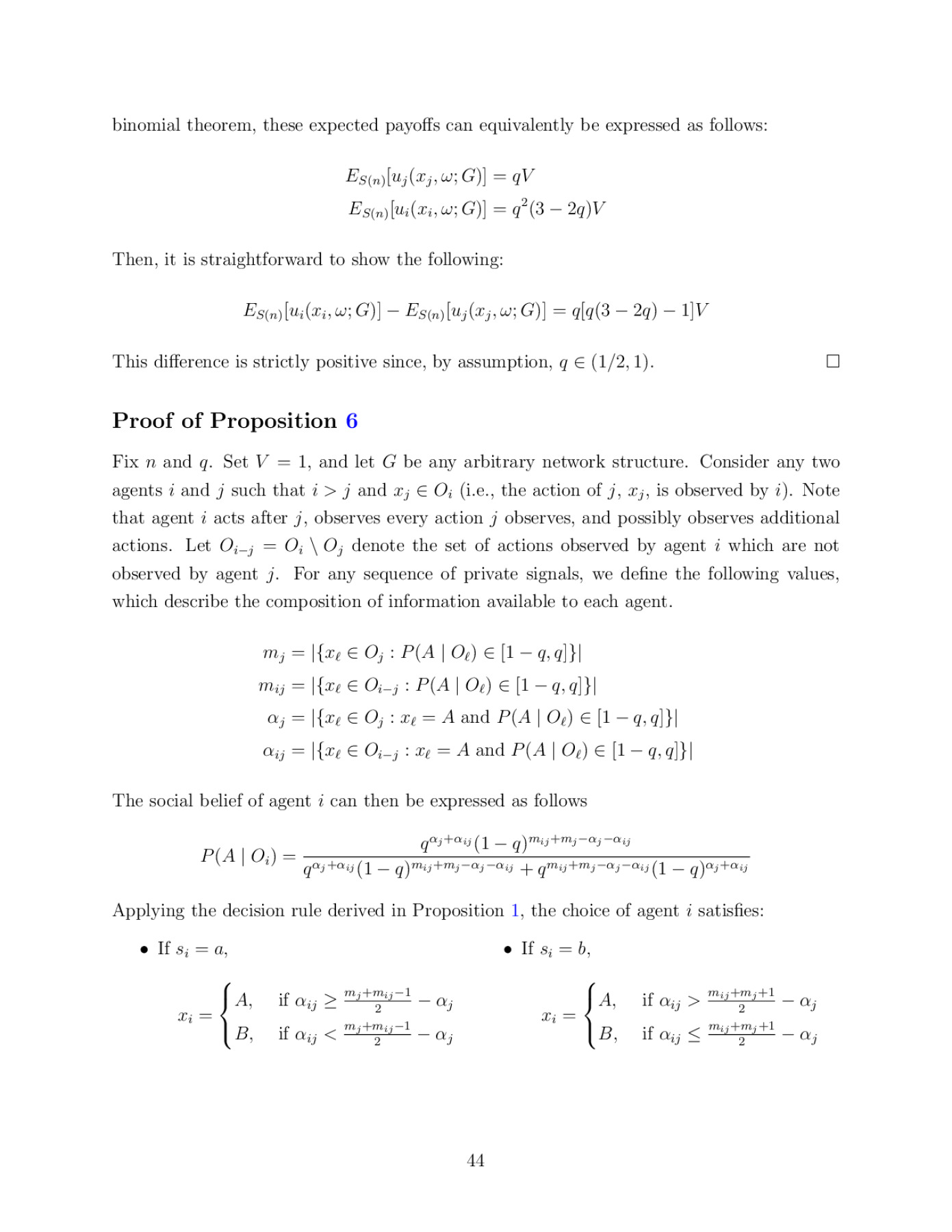


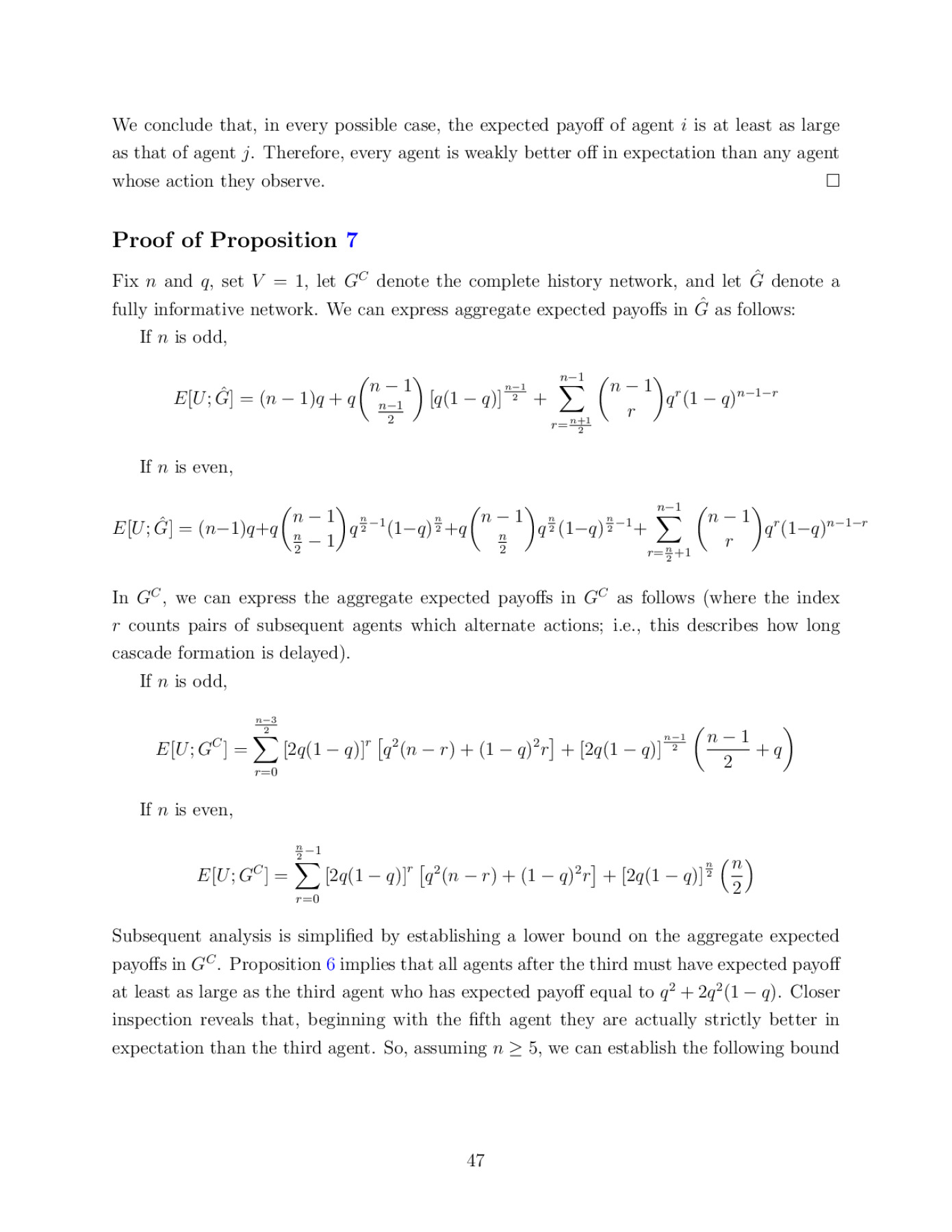
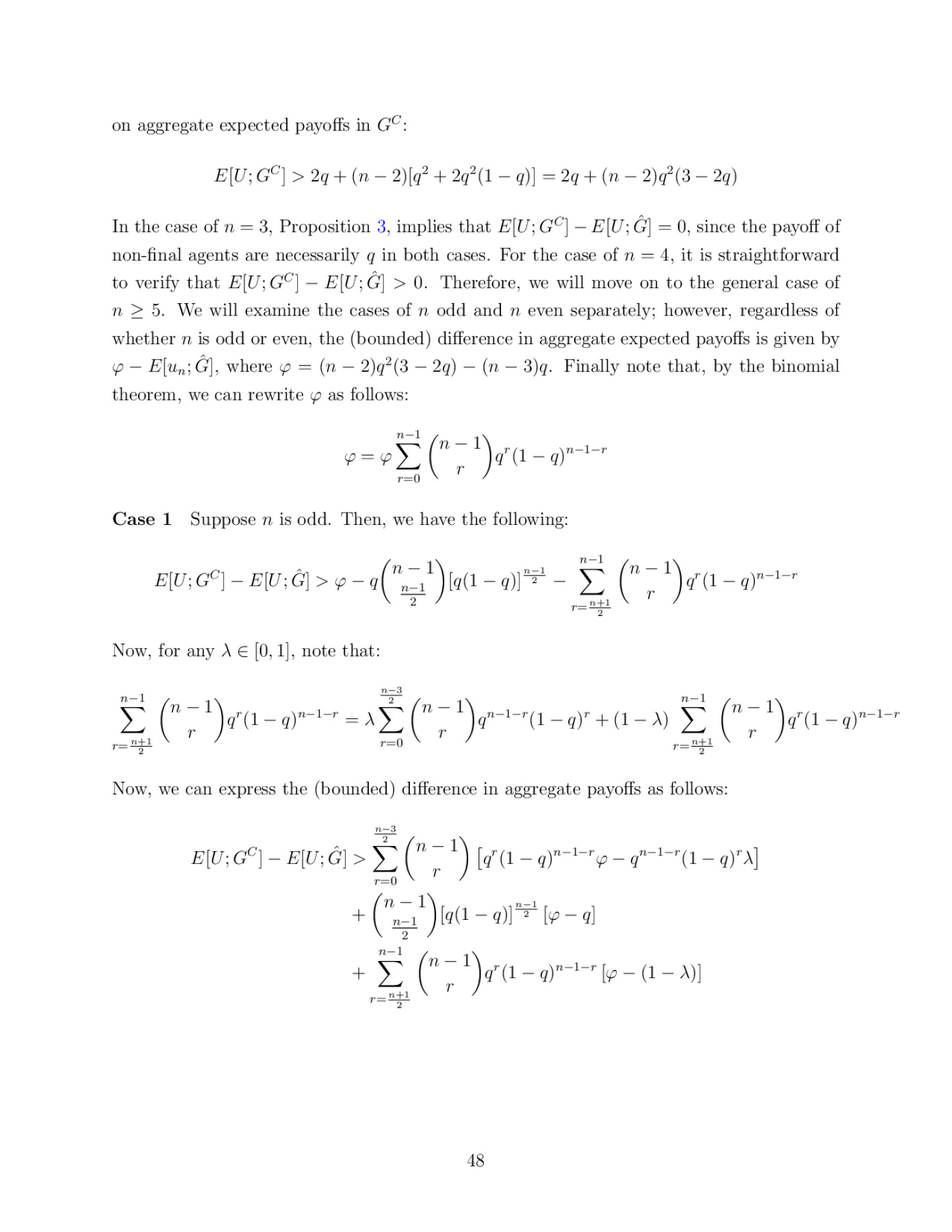

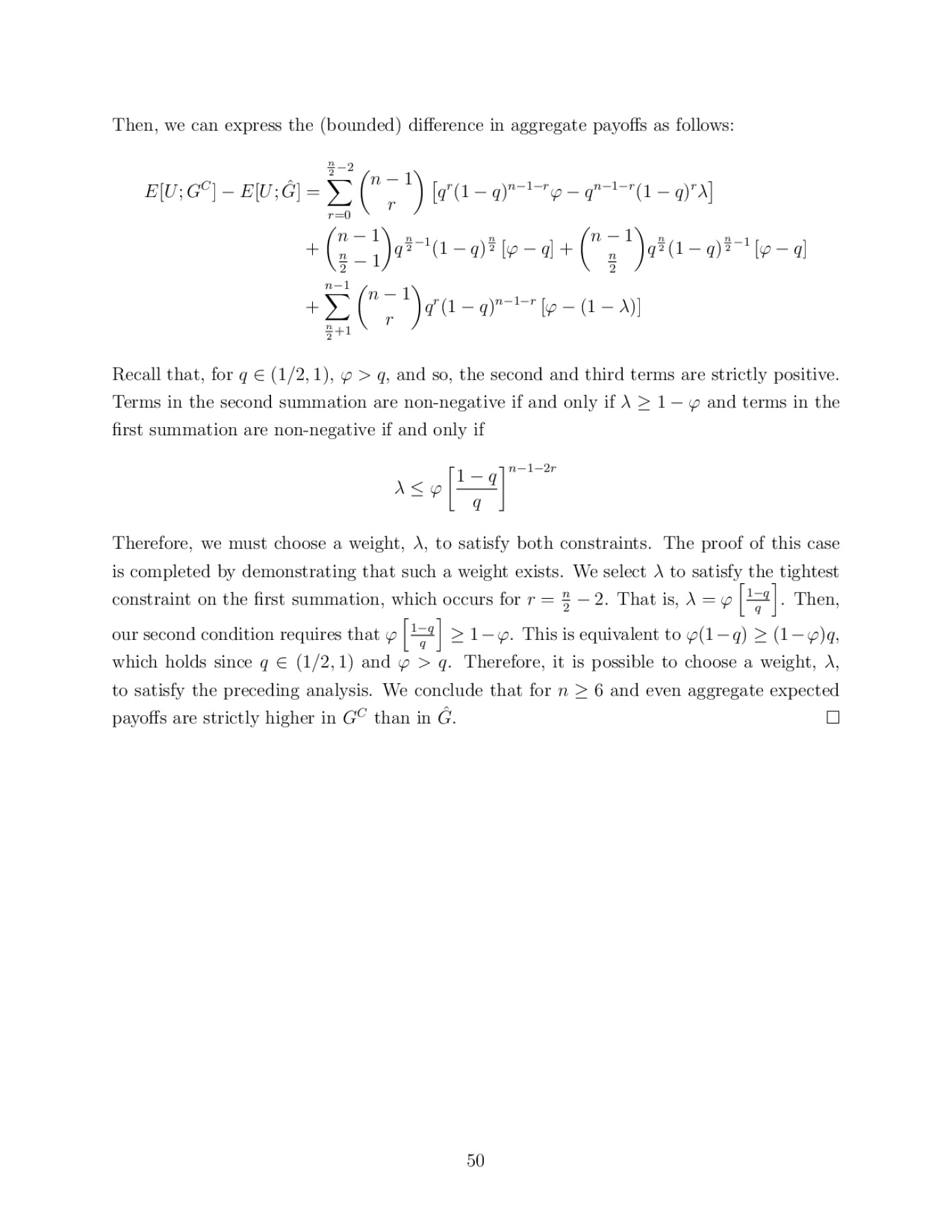
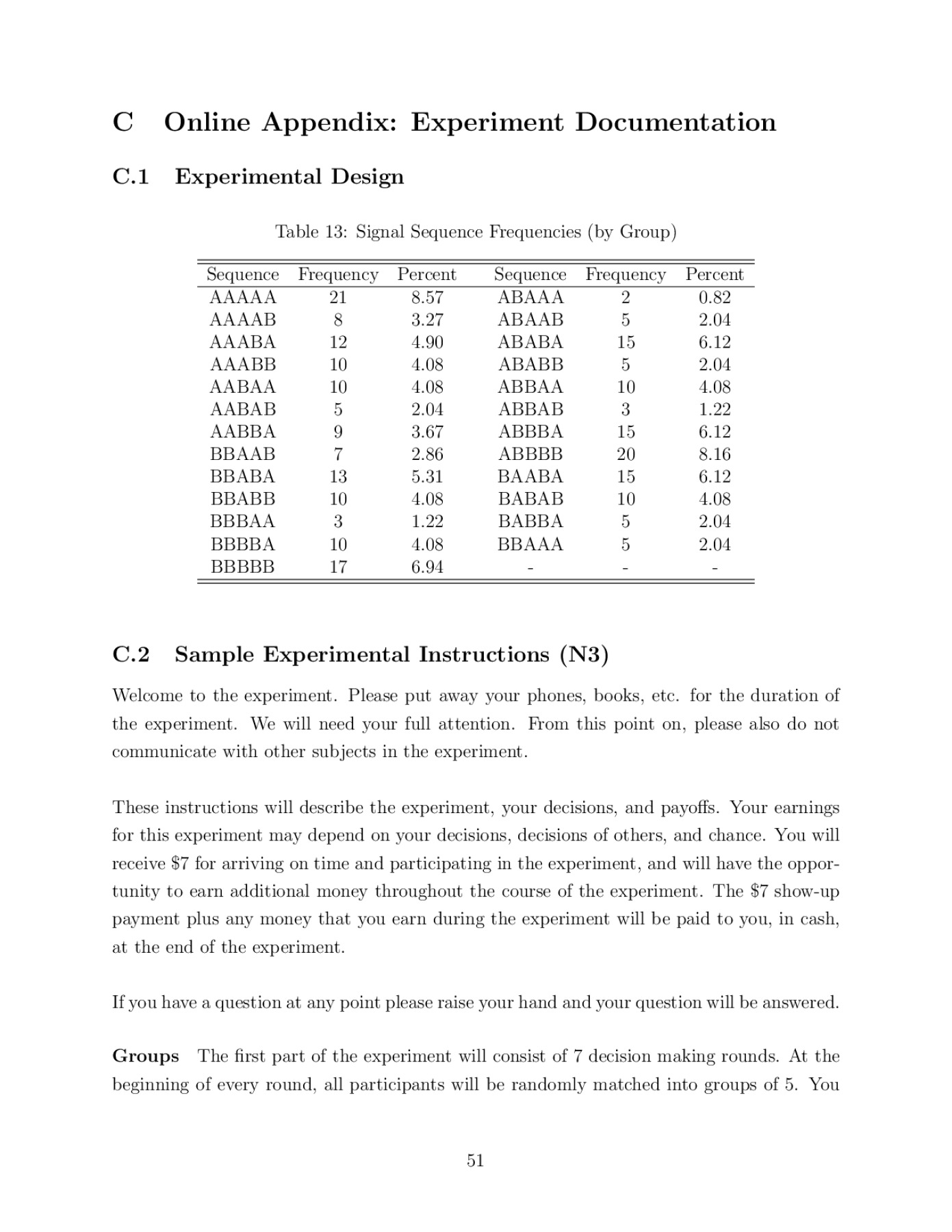

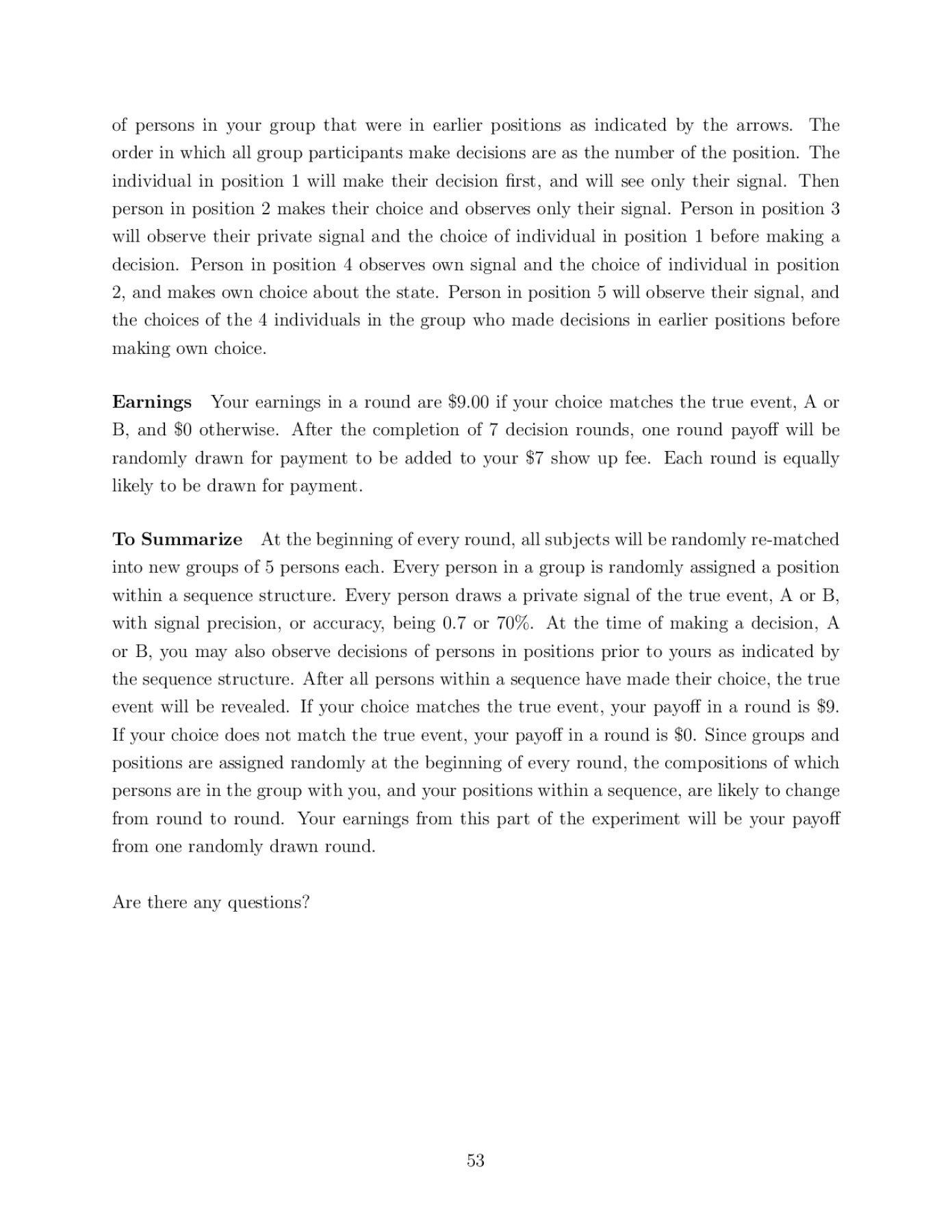
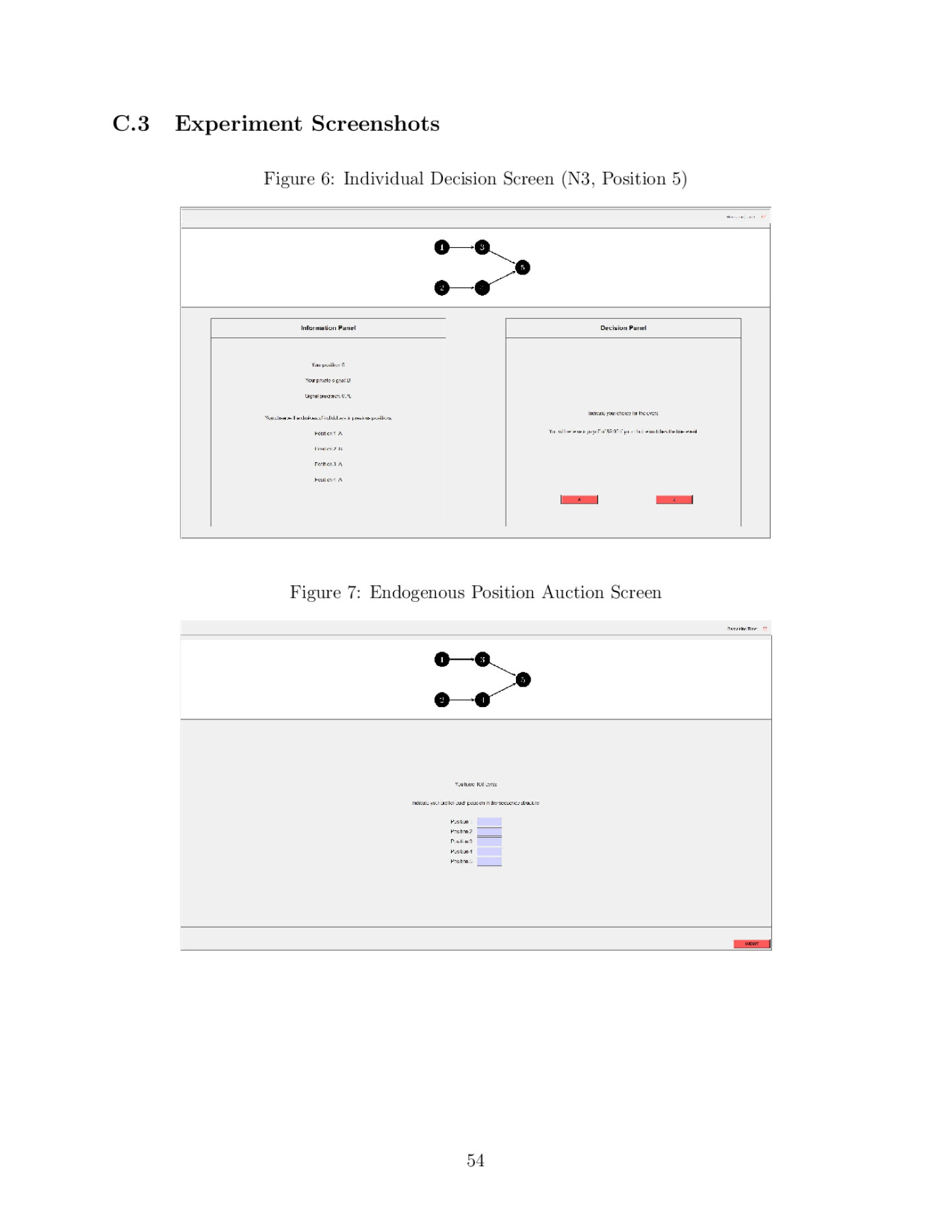