2024 European Winter Meeting, Palma de Majorca, Spain: December, 2024
The heterogeneous impact of the EU-Canada agreement with causal machine learning
Lionel Fontagné, Francesca Micocci, Armando Rungi
This paper introduces a causal machine learning approach to investigate the impact of the EU-Canada Comprehensive Economic Trade Agreement (CETA). We propose a matrix completion algorithm on French customs data to obtain multidimensional counterfactuals at the firm, product and destination levels. We find a small but significant positive impact on average at the product-level intensive margin. On the other hand, the extensive margin shows product churning due to the treaty beyond regular entry-exit dynamics: one product in eight that was not previously exported substitutes almost as many that are no longer exported. When we delve into the heterogeneity, we find that the effects of the treaty are higher for products at a comparative advantage. Focusing on multiproduct firms, we find that they adjust their portfolio in Canada by reallocating towards their first and most exported product due to increasing local market competition after trade liberalization. Finally, multidimensional counterfactuals allow us to evaluate the general equilibrium effect of the CETA. Specifically, we observe trade diversion, as exports to other destinations are re-directed to Canada.
Preview
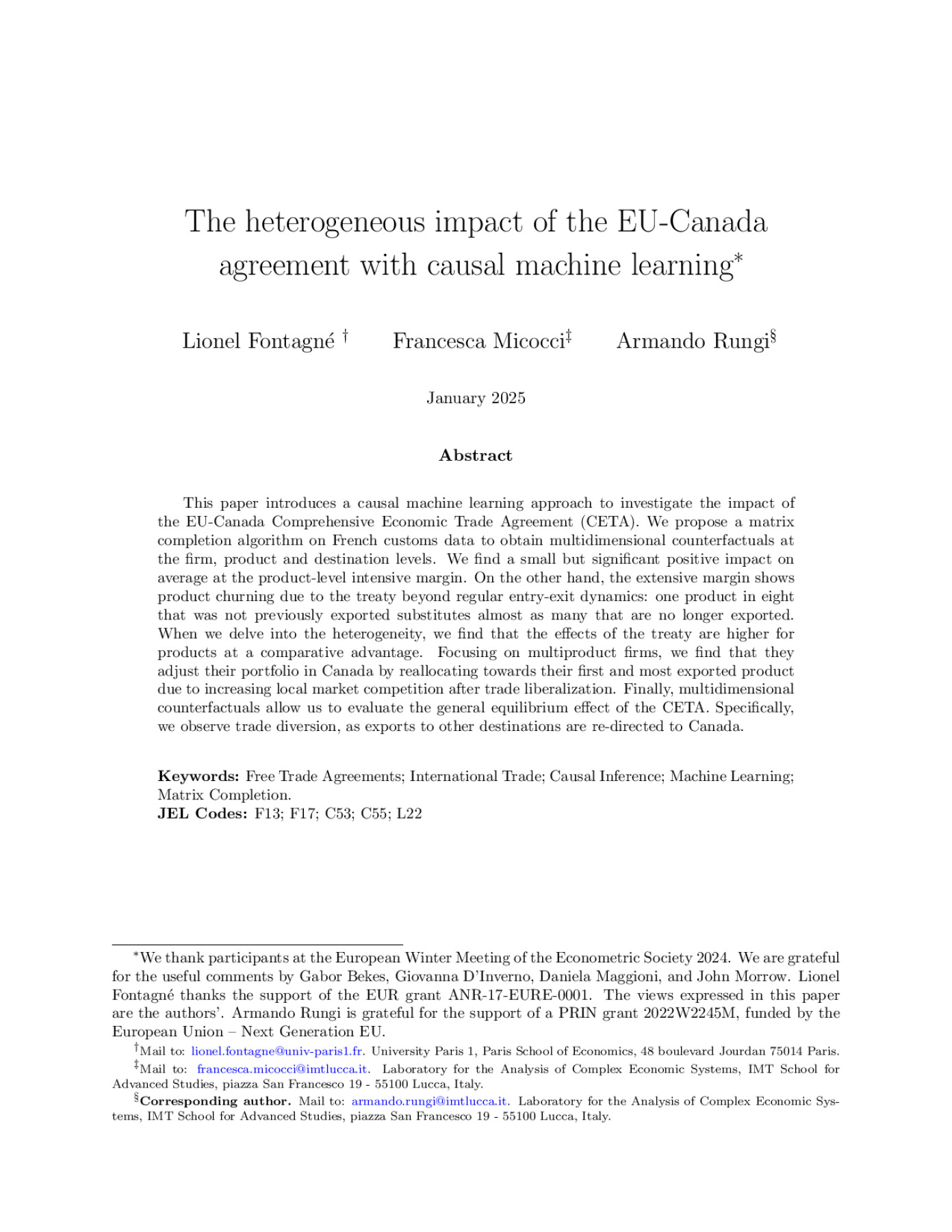















































